Adding an Aquatic Prey Fish Module within the Everglades Vulnerability Analysis Modeling Framework
The USGS Joint Ecosystem Modeling team will use Bayesian networks to build a spatially explicit Everglades Vulnerability Analysis module based on current knowledge and existing data on fish density and biomass trends on the landscape.
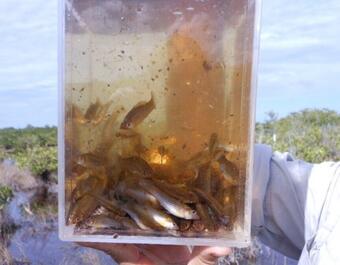
The Science Issue and Relevance: Ecological modeling is important in facilitating evaluation and assessment of management actions targeted toward ecosystem restoration goals. The value of ecological modeling for restoration planning is strongest when model outputs are designed to meet the needs of decision makers. Joint Ecosystem Modeling (JEM), led by the U.S. Geological Survey (USGS), is a partnership among federal and state agencies, universities, and other organizations that provides ecological model output tailored to management decisions. Many models have been developed to predict species population responses, habitat suitability, and forecast hydrologic conditions in the Greater Everglades region. One such model is the Everglades Vulnerability Analysis (EVA), a series of connected Bayesian networks that model the landscape-scale response of indicators of Everglades ecosystem health to changes in hydrology and salinity. These indicators include vegetation type, size of wading bird colonies, presence of alligator nests, and sawgrass peat dynamics. The EVA tool was designed so that new modules representing additional indicators of Everglades ecosystem health could be developed and connected to current modules to further the tool’s system-wide assessment capabilities. This project will add a new module to EVA describing the changes in fish density and biomass across the Everglades system in both the wet and dry seasons.
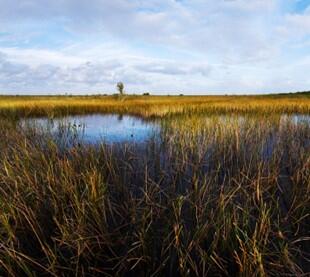
Methodology for Addressing the Issue: We will use Bayesian networks to build a spatially explicit EVA module based on current knowledge and existing data on fish density and biomass trends on the landscape. Everglades fish experts will be consulted to determine the best model parameterizations and to ensure the output reasonably reflects the ecological trends and mechanisms behind fish density and biomass fluctuations. We will test the module’s ability to accurately predict variables based on monitoring data and test the model’s sensitivity to changes in salinity and hydrologic inputs.
Future Steps: This addition to the EVA tool will be used by restoration planners, natural resource managers, and decision-makers to assess the potential impacts of changing environmental conditions and restoration on the system-wide trends of prey fish density and biomass within the Everglades landscape. The outputs from this tool are directly tied to system-wide Everglades monitoring efforts and can be used to assess whether future predictions are aligning with on-the-ground observations as restoration and environmental change progresses.
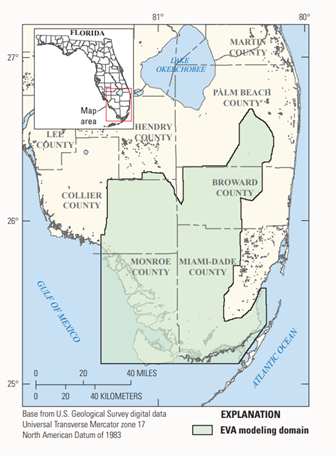
Joint Ecosystem Modeling: Cape Sable Seaside Sparrow Helper
Joint Ecosystem Modeling: Wader Distribution & Evaluation Modeling (WADEM)
Joint Ecosystem Modeling: Cape Sable Seaside Sparrow Marl Prairie Indicator
Joint Ecosystem Modeling: EverSnail
Joint Ecosystem Modeling: Alligator Production Probability Model
Joint Ecosystem Modeling: Greater Everglades Modeling Decision Support Tools
Advanced Technological Solutions in Support of Greater Everglades Priority Ecosystem Science: Joint Ecosystem Modeling (JEM)
The USGS Joint Ecosystem Modeling team will use Bayesian networks to build a spatially explicit Everglades Vulnerability Analysis module based on current knowledge and existing data on fish density and biomass trends on the landscape.
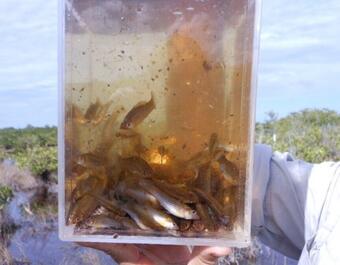
The Science Issue and Relevance: Ecological modeling is important in facilitating evaluation and assessment of management actions targeted toward ecosystem restoration goals. The value of ecological modeling for restoration planning is strongest when model outputs are designed to meet the needs of decision makers. Joint Ecosystem Modeling (JEM), led by the U.S. Geological Survey (USGS), is a partnership among federal and state agencies, universities, and other organizations that provides ecological model output tailored to management decisions. Many models have been developed to predict species population responses, habitat suitability, and forecast hydrologic conditions in the Greater Everglades region. One such model is the Everglades Vulnerability Analysis (EVA), a series of connected Bayesian networks that model the landscape-scale response of indicators of Everglades ecosystem health to changes in hydrology and salinity. These indicators include vegetation type, size of wading bird colonies, presence of alligator nests, and sawgrass peat dynamics. The EVA tool was designed so that new modules representing additional indicators of Everglades ecosystem health could be developed and connected to current modules to further the tool’s system-wide assessment capabilities. This project will add a new module to EVA describing the changes in fish density and biomass across the Everglades system in both the wet and dry seasons.
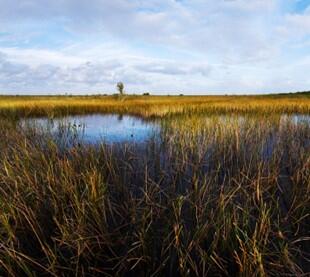
Methodology for Addressing the Issue: We will use Bayesian networks to build a spatially explicit EVA module based on current knowledge and existing data on fish density and biomass trends on the landscape. Everglades fish experts will be consulted to determine the best model parameterizations and to ensure the output reasonably reflects the ecological trends and mechanisms behind fish density and biomass fluctuations. We will test the module’s ability to accurately predict variables based on monitoring data and test the model’s sensitivity to changes in salinity and hydrologic inputs.
Future Steps: This addition to the EVA tool will be used by restoration planners, natural resource managers, and decision-makers to assess the potential impacts of changing environmental conditions and restoration on the system-wide trends of prey fish density and biomass within the Everglades landscape. The outputs from this tool are directly tied to system-wide Everglades monitoring efforts and can be used to assess whether future predictions are aligning with on-the-ground observations as restoration and environmental change progresses.
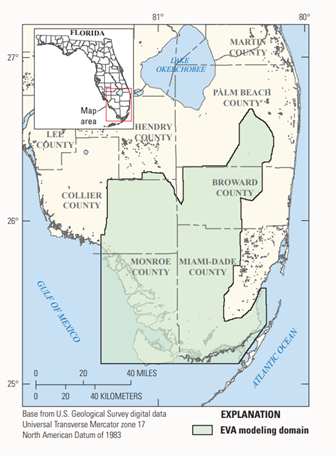