The U.S. Geological Survey (USGS) 3D Elevation Program (3DEP) provides high accuracy, high-resolution (HR) elevation data for the United States.
Larry Stanislawski
Lawrence (Larry) V. Stanislawski is a Research Cartographer for the Center of Excellence for Geospatial Information Science (CEGIS). His work focuses on generalization and multiscale representation that support or enable automated mapping and science investigations using geospatial data, particularly the National Map datasets.
Larry received his B.S. in Forest Resources and Conservation and his M.S. in Forest Remote Sensing from the University of Florida. He continued studying in the Surveying and Mapping Program at the University of Florida and performed research on GIS data accuracy and on high precision surveying with Global Position Systems (GPS). Prior to his work with the U.S. Geological Survey, Larry worked in various geoscience research and consultant positions, and as a GIS developer with the Army Corps of Engineers in Jacksonville, Florida. In 1998, he and his family moved to Rolla, Missouri where he began as a GIS Developer with National Geospatial Technical Operations Center leading development of automated systems to build the high-resolution National Hydrography Dataset (NHD) with conflation of medium resolution NHD data. During this time, he also designed and taught a Geomatics course at Missouri University of Science and Technology. Larry began working as a CEGIS research scientist in 2011. Larry’s research includes machine learning and high-performance computing to extract, validate, and generalize hydrography and other features using high resolution elevation and remotely sensed data, such as lidar from the 3D Elevation Program.
Science and Products
At what scales does a river meander? Scale-specific sinuosity (S3) metric for quantifying stream meander size distribution
Generalization quality metrics to support multiscale mapping: Hausdorff and average distance between polylines
Transferring deep learning models for hydrographic feature extraction from IfSAR data in Alaska
Automated mapping of culverts, bridges, and dams
Comparing line feature morphology with scale specific sinuosity distributions: A modified earth mover’s distance
Scaling-up deep learning predictions of hydrography from IfSAR data in Alaska
Weakly supervised spatial deep learning for Earth image segmentation based on imperfect polyline labels
GeoAI in the US Geological Survey for topographic mapping
Extensibility of U-net neural network model for hydrographic feature extraction and implications for hydrologic modeling
Watersheds and drainage networks
The 4th paradigm in multiscale data representation: Modernizing the National Geospatial Data Infrastructure
An attention U-Net model for detection of fine-scale hydrologic streamlines
Non-USGS Publications**
**Disclaimer: The views expressed in Non-USGS publications are those of the author and do not represent the views of the USGS, Department of the Interior, or the U.S. Government.
Automated accuracy and quality assessment tools (AQAT = “a cat”) for generalized geospatial data
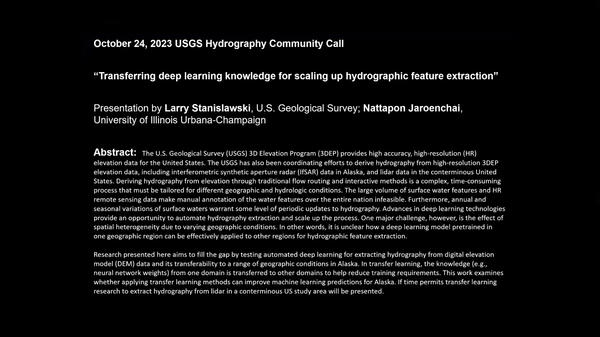
The U.S. Geological Survey (USGS) 3D Elevation Program (3DEP) provides high accuracy, high-resolution (HR) elevation data for the United States.
Science and Products
At what scales does a river meander? Scale-specific sinuosity (S3) metric for quantifying stream meander size distribution
Generalization quality metrics to support multiscale mapping: Hausdorff and average distance between polylines
Transferring deep learning models for hydrographic feature extraction from IfSAR data in Alaska
Automated mapping of culverts, bridges, and dams
Comparing line feature morphology with scale specific sinuosity distributions: A modified earth mover’s distance
Scaling-up deep learning predictions of hydrography from IfSAR data in Alaska
Weakly supervised spatial deep learning for Earth image segmentation based on imperfect polyline labels
GeoAI in the US Geological Survey for topographic mapping
Extensibility of U-net neural network model for hydrographic feature extraction and implications for hydrologic modeling
Watersheds and drainage networks
The 4th paradigm in multiscale data representation: Modernizing the National Geospatial Data Infrastructure
An attention U-Net model for detection of fine-scale hydrologic streamlines
Non-USGS Publications**
**Disclaimer: The views expressed in Non-USGS publications are those of the author and do not represent the views of the USGS, Department of the Interior, or the U.S. Government.
Automated accuracy and quality assessment tools (AQAT = “a cat”) for generalized geospatial data
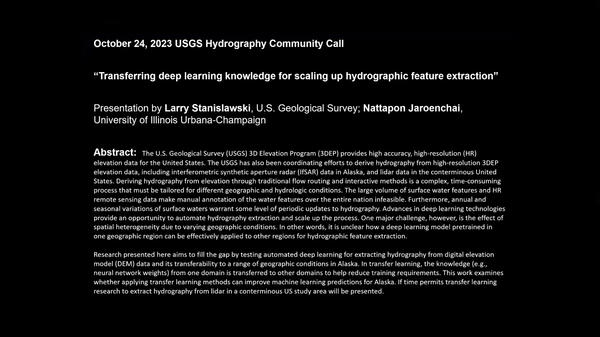
The U.S. Geological Survey (USGS) 3D Elevation Program (3DEP) provides high accuracy, high-resolution (HR) elevation data for the United States.
The U.S. Geological Survey (USGS) 3D Elevation Program (3DEP) provides high accuracy, high-resolution (HR) elevation data for the United States.