Hierarchical Models for Estimating Abundance and Occurrence of Individual Species Active
USGS is working to develop hierarchical models that will help analyze population-level surveys and sampling protocols, including presence-absence, point-count, multiple observer, removal, and capture-recapture.
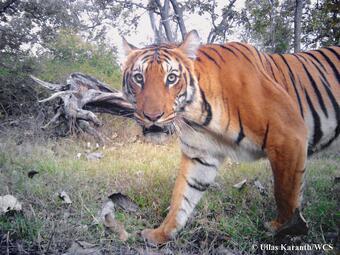
The Science Issue and Relevance: Investigations of populations of individual species often require estimates of abundance or occurrence. These estimates may be used to monitor spatial or temporal changes in the population or to determine whether relationships exist between individuals and one or more environmental characteristics (e.g., to identify habitat). A common difficulty in these studies is that individuals which are available to be sampled often go undetected; thus, the number of individuals observed in a survey provides a negatively biased index of abundance. These biases are important because rates of detection can vary among collection times, locations, observers, and even among individual members of the population (say, owing to differences in animal behavior or coloration). Therefore, accounting for the effects of imperfect detection is crucial in the analysis of population survey data.
Methodology for Addressing the Issue: The focus of this research is to develop statistical models for the analysis of surveys of natural populations. A hierarchical approach is adopted wherein an observation model, which accounts for the sampling process and for imperfect detection, is augmented with a model of species occurrences or abundances. The latter component is generally tailored to exploit site-level covariates that are thought to be informative of species occurrence or abundance.
Future Steps: Hierarchical models have been developed for a variety of population-level surveys and sampling protocols, including presence-absence surveys, point-count surveys, multiple- observer surveys, removal surveys, and capture-recapture surveys. Surveys of unmarked individuals continue to pose challenges for analysis that motivate the development of new statistical models. Some particularly difficult examples include analysis of presence-only data, analysis of rare species surveyed by adaptive-sampling protocols, and analysis of an invasive species during and after the initial colonization of the species.
Related Project(s): Hierarchical Models for Computing Inferences About Species Assemblages Subject to Imperfect Detection
Publications:
- Royle, J.A. and R.M. Dorazio. 2012. Parameter-expanded data augmentation for Bayesian analysis of capture-recapture models. Journal of Ornithology 152 (Supplement 2): S521--S537.
- Shirley, M.H., R.M. Dorazio, E. Abassery, A. Elhady, M.S. Mekki, and H.H. Asran. 2012. A sampling design and model for estimating abundance of Nile crocodiles while accounting for heterogeneity of detectability of multiple observers. Journal of Wildlife Management 76: 966--975.
- Pacifici, K., R.M. Dorazio, and M.J. Conroy. 2012. A two-phase sampling design for increasing detections of rare species in occupancy surveys. Methods in Ecology and Evolution 3: 721--730.
- Dorazio, R.M. 2012. Predicting the geographic distribution of a species from presence-only data subject to detection errors. Biometrics 68: 1303--1312.
- Dorazio, R.M. and D. Taylor Rodriguez. 2012. A Gibbs sampler for Bayesian analysis of site-occupancy data. Methods in Ecology and Evolution 3: 1093--1098.
- Dorazio, R.M., J. Martin, and H.H. Edwards. 2013. Estimating abundance while accounting for rarity, correlated behavior of animals, and other sources of variation in counts. Ecology 94: 1472--1478.
- Dorazio, R.M. 2013. Bayes and empirical Bayes estimators of abundance and density from spatial capture-recapture data. PLoS ONE 8: e84017.
- Johnson, F.A., R.M. Dorazio, T.D. Castellon, J. Martin, J.O. Garcia, and J.D. Nichols. 2014. Tailoring point counts for inference about avian density: dealing with nondetection and availability. Natural Resource Modeling 27: 163--177.
- Dorazio, R.M. 2014. Accounting for imperfect detection and survey bias in statistical analysis of presence-only data. Global Ecology and Biogeography 23: 1472--1484.
- Hunter, M.E., S.J. Oyler-McCance, R.M. Dorazio, J.A. Fike, B.J. Smith, C.T. Hunter, R.N. Reed, and K.M. Hart.
Below are other science projects associated with this project.
Hierarchical Models for Computing Inferences About Species Assemblages Subject to Imperfect Detection
Below are publications associated with this project.
Accounting for imperfect detection and survey bias in statistical analysis of presence-only data
Tailoring point counts for inference about avian density: dealing with nondetection and availability
Bayes and empirical Bayes estimators of abundance and density from spatial capture-recapture data
Estimating abundance while accounting for rarity, correlated behavior, and other sources of variation in counts
A sampling design and model for estimating abundance of Nile crocodiles while accounting for heterogeneity of detectability of multiple observers
A two-phase sampling design for increasing detections of rare species in occupancy surveys
Parameter-expanded data augmentation for Bayesian analysis of capture-recapture models
Predicting the geographic distribution of a species from presence-only data subject to detection errors
A Gibbs sampler for Bayesian analysis of site-occupancy data
USGS is working to develop hierarchical models that will help analyze population-level surveys and sampling protocols, including presence-absence, point-count, multiple observer, removal, and capture-recapture.
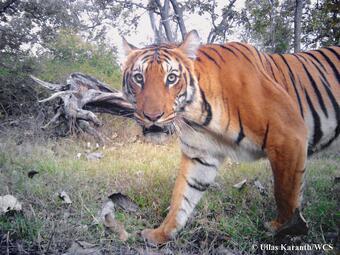
The Science Issue and Relevance: Investigations of populations of individual species often require estimates of abundance or occurrence. These estimates may be used to monitor spatial or temporal changes in the population or to determine whether relationships exist between individuals and one or more environmental characteristics (e.g., to identify habitat). A common difficulty in these studies is that individuals which are available to be sampled often go undetected; thus, the number of individuals observed in a survey provides a negatively biased index of abundance. These biases are important because rates of detection can vary among collection times, locations, observers, and even among individual members of the population (say, owing to differences in animal behavior or coloration). Therefore, accounting for the effects of imperfect detection is crucial in the analysis of population survey data.
Methodology for Addressing the Issue: The focus of this research is to develop statistical models for the analysis of surveys of natural populations. A hierarchical approach is adopted wherein an observation model, which accounts for the sampling process and for imperfect detection, is augmented with a model of species occurrences or abundances. The latter component is generally tailored to exploit site-level covariates that are thought to be informative of species occurrence or abundance.
Future Steps: Hierarchical models have been developed for a variety of population-level surveys and sampling protocols, including presence-absence surveys, point-count surveys, multiple- observer surveys, removal surveys, and capture-recapture surveys. Surveys of unmarked individuals continue to pose challenges for analysis that motivate the development of new statistical models. Some particularly difficult examples include analysis of presence-only data, analysis of rare species surveyed by adaptive-sampling protocols, and analysis of an invasive species during and after the initial colonization of the species.
Related Project(s): Hierarchical Models for Computing Inferences About Species Assemblages Subject to Imperfect Detection
Publications:
- Royle, J.A. and R.M. Dorazio. 2012. Parameter-expanded data augmentation for Bayesian analysis of capture-recapture models. Journal of Ornithology 152 (Supplement 2): S521--S537.
- Shirley, M.H., R.M. Dorazio, E. Abassery, A. Elhady, M.S. Mekki, and H.H. Asran. 2012. A sampling design and model for estimating abundance of Nile crocodiles while accounting for heterogeneity of detectability of multiple observers. Journal of Wildlife Management 76: 966--975.
- Pacifici, K., R.M. Dorazio, and M.J. Conroy. 2012. A two-phase sampling design for increasing detections of rare species in occupancy surveys. Methods in Ecology and Evolution 3: 721--730.
- Dorazio, R.M. 2012. Predicting the geographic distribution of a species from presence-only data subject to detection errors. Biometrics 68: 1303--1312.
- Dorazio, R.M. and D. Taylor Rodriguez. 2012. A Gibbs sampler for Bayesian analysis of site-occupancy data. Methods in Ecology and Evolution 3: 1093--1098.
- Dorazio, R.M., J. Martin, and H.H. Edwards. 2013. Estimating abundance while accounting for rarity, correlated behavior of animals, and other sources of variation in counts. Ecology 94: 1472--1478.
- Dorazio, R.M. 2013. Bayes and empirical Bayes estimators of abundance and density from spatial capture-recapture data. PLoS ONE 8: e84017.
- Johnson, F.A., R.M. Dorazio, T.D. Castellon, J. Martin, J.O. Garcia, and J.D. Nichols. 2014. Tailoring point counts for inference about avian density: dealing with nondetection and availability. Natural Resource Modeling 27: 163--177.
- Dorazio, R.M. 2014. Accounting for imperfect detection and survey bias in statistical analysis of presence-only data. Global Ecology and Biogeography 23: 1472--1484.
- Hunter, M.E., S.J. Oyler-McCance, R.M. Dorazio, J.A. Fike, B.J. Smith, C.T. Hunter, R.N. Reed, and K.M. Hart.
Below are other science projects associated with this project.
Hierarchical Models for Computing Inferences About Species Assemblages Subject to Imperfect Detection
Below are publications associated with this project.