Standardized Emergency Stabilization and Rehabilitation Monitoring Protocols (ES&R)
Fire rehabilitation programs have existed within federal agencies since the early 1960s. The U.S. Forest Service and Bureau of Land Management are the largest users of emergency stabilization and rehabilitation (ES&R) funds, but these agencies only sporadically implement proposed monitoring and rarely use common protocols. As a result, it is impossible to draw scientifically credible conclusions regarding the effectiveness of ES&R projects. Additionally, the data that does exist are stored in field office files and are often not available for other managers to use when planning new ES&R treatments.
A common monitoring strategy helps address concerns through
- Implementation of a common approach and methodology to assess the effectiveness of ES&R projects region-wide
- Use of field based computers to enter data in a standard format that is subequently uploaded to a centralized database
- Analysis of collected data at regular intervals to determine overall success and to identify factors involved in successful treatments
By following this strategy, managers can ultimately determine the effectiveness of post-fire rehabilitation activities region-wide.
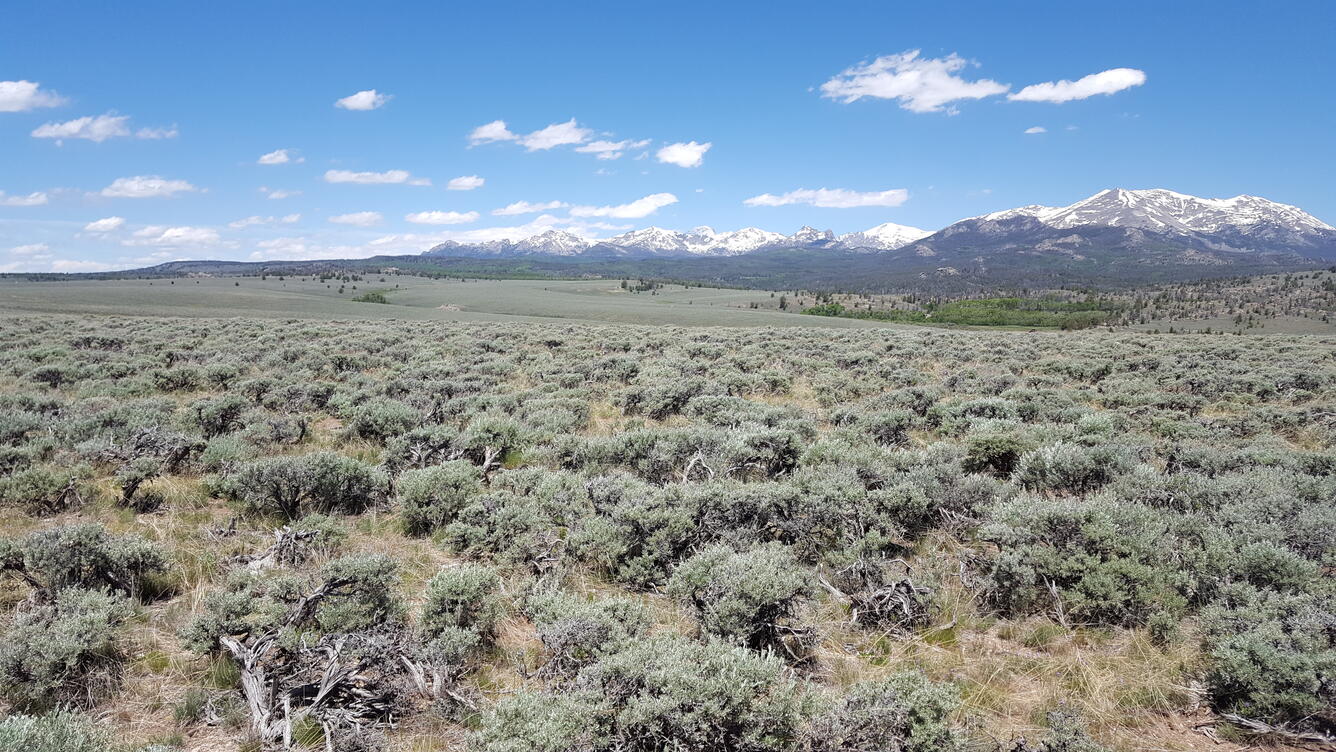
The Approach
We reviewed seven vegetation monitoring manuals produced by federal agencies to determine which elements would be appropriate for evaluating ES&R treatment success.
Monitoring Manual for Grasslands, Shrublands, and Savanna Ecosystems (Volumes 1 and 2)
Herrick, J.E., Van Zee, J.W., Havstad, K.M., Burkett, L.M., Whitford, W.G. 2005a. Monitoring Manual For Grassland, Shrubland and Savanna Ecosystems. Volume 1: Quick Start. USDA-ARS Jornada Experimental Range. 36 p.
Herrick, J.E., Van Zee, J.W., Havstad, K.M, Burkett, L.M., Whitford, W.G. 2005b. Monitoring Manual For Grassland, Shrubland and Savanna Ecosystems. Volume 2: Design, Supplementary Methods and Interpretation. USDA-ARS Jornada Experimental Range. 200 p.
Measuring and monitoring plant populations
Elzinga, C.L., Salzer, D.W., and Willoughby, J.W. 1998. Measuring and Monitoring Plant Populations. USDI Bureau of Land Management Technical Reference 1730-1. 492p.
Sampling vegetation attributes
Interagency Technical Reference. 1999. Sampling Vegetation Attributes. BLM Technical Reference 1734-4. 158 p.
Fire Effects Monitoring and Inventory Protocol (FIREMON)
Lutes, Duncan C., Keane, Robert, E., Caratti, John. F., Key, Carl H., Benson, Nathan C., Sutherland, Steve, Gangi, Larry J., 2006. FIREMON: Fire Effects Monitoring and Inventory System. Gen. Tech. Rep. RMRS-GTR-164-CD. For Collins, CO: U.S. Department of Agriculture, Forest Service, Rocky Mountain Research Station. 1 CD. 400 p.
USDI National Park Service. 2003. Fire Monitoring Handbook. Boise, ID: Fire Management program Center, National Interagency Fire Center. 274 p.
Fuel and Fire Effects Monitoring Guide
USDI Fish and Wildlife Service. 1999. Fuel and Fire Effects Monitoring Guide.
Land Condition Trend Analysis
U.S. Army Sustainable Range Program, 2006. RTLA Technical Reference Manual: Ecological Monitoring on Military Lands.
The following are descriptions of program design elements found within the reviewed monitoring manuals that are suitable for use in evaluating ES&R treatment success.
Objectives
All seven manuals included objectives as an important element of a monitoring program. Several manuals also provide good descriptions of how to formulate objectives.
Measuring and Monitoring Plant Populations inidcates both management and sampling objectives should be written for any project. Management objectives are “clearly articulated descriptions of a measurable standard, desired state, threshold value, amount of change, or trend that you are striving to achieve for a particular plant population or habitat characteristics.”
Well-defined management objectives in a monitoring program perform two functions.
- They establish a standard to measure the degree of success, and
- They determine the appropriate indicators to measure.
A standard protocol can then be followed for the measurement of each indicator. Thus, data collection activities are directly related to management objectives. Sampling objectives should be paired with each management objective and specify the desired confidence level, precision - confidence interval width, level of type II error, or detectable change for the sampling effort.
Stratification
Five of the seven monitoring manuals describe stratification. Stratification is the partitioning of treatment areas to reduce variation and increase precision of sampling efforts. Areas that may respond differently to ES&R treatments such as different soil types or ecological sites are good candidates for strata.
Rules for stratification of treatment areas into monitoring units should be created during the planning stage of an ES&R project. Stratification can be undertaken concurrently with or after identification of treatment areas. In many cases, stratification is completed as a by-product of treatment planning such as assigning different seed mixes to sites with different characteristics or potentials.
Background information on the treatment area is essential for stratification. A variety of GIS data are useful for delineating monitoring units, including digital elevation models (DEMs), fire perimeters, proposed and actual treatment areas, soils (if available), roads, and land-use information. Monitoring units can be derived with GIS software, such as ArcGIS, based on the available information and the specifics of the project.
- Shapefiles for ecological sites may be the preferred initial strata, if available.
- If only soils are available, then the site can be divided initially into soil strata separately to reduce variation and increase monitoring efficiency.
- If only soil surveys are available, but soil-to-ecological site correlations are known, then differing soils that correlate to the same ecological site may be combined into the same strata.
- Slope classes can be generated from DEMs when seedings will occur over a large range of slopes.
- Areas that are not likely to be seeded due to topography can be excluded from the monitoring unit using DEMs.
Stratification of the area for both treatments and monitoring can be accomplished using a defined set of variables such as slope, aspect, elevation, treatment type, minimum size, soil type, or ecological site.
Descriptions of monitoring units should be included in monitoring plans so that the scope of inference is known. For example:
- Monitoring unit 1 includes all areas of less than 20 percent slope within soil type A in the native seed mix treatment.
- Monitoring unit 2 includes all areas equal to or greater than 20 percent slope within soil type A in the native seed mix treatment.
- Monitoring unit 3 includes all areas of less than 20 percent slope within the non-native seed mix treatment.
Similar methods of stratification used on different projects will facilitate comparisons among those projects and aid region-wide assessments of ES&R treatment effectiveness. Additional information on stratification can be found in the Fire Monitoring Handbook, Fire Effects Monitoring and Inventory Protocol, and the Monitoring Manual for Grassland, Shrubland, and Savanna Ecosystems.
Control Plots
Control plots are locations within a proposed treatment area that are established prior to and avoided when treatments are implemented. They provide important information on natural recovery that can be used to determine whether or not treatments were necessary in the first place. This is especially useful given limited resources for implementing large projects in severe fire years. The Fire Monitoring Handbook states that control areas should be used when attributing a particular effect to the applied treatment. The goal is to show that the treatment caused the observed change.
Prior to applying treatments, a minimum of three control plots should be randomly placed within each monitoring unit. Control plots should not be placed in adjacent untreated areas because they were not proposed to be treated and are therefore different from those areas that were proposed to be treated. Since adjacent areas are most likely different from the area being treated, they make poor controls.
Control plots may not be practical in all situations such as in cases where life or property are threatened. These situations often occur when the areas to be treated are on slopes above developments and it is not possible to leave some areas untreated. However, controls should be used whenever possible because they provide the best measure of natural regeneration and ES&R treatment success.
Random Sampling
Random sampling ensures that monitoring data are unbiased and representative of the monitoring unit. While this may be time-consuming, it is essential for defensible monitoring data and success determinations. Without random sampling, criticism may include that the samples only came from areas where the treatments were effective, or that data were biased by the site-selection process. In addition, data that are not derived from random sampling are only valid for the plot at which they were collected and cannot be used to infer to the rest of the treatment area.
There are several different methods of random sampling that can be used to monitor ES&R treatments: simple, systematic, restricted, or two-stage random sampling. One of these methods should be used to enable statistical inference over as much of the treated area as possible. To generate random samples, there are several options, including grids placed over maps or GIS random-point generators.
Rejection criteria should be defined and procedures established if random plot locations occur in areas that cannot be seeded, such as roads, rocky outcrops, steep slopes, streams, or other features. In some cases it may be possible to relocate the plot nearby in a random direction. Or, it may be necessary to move to the next randomly generated point.
Data Quality
After collecting monitoring data, it is helpful to determine how well it can assess ES&R treatment success. Parameters that should be examined are confidence intervals, sample-size estimates, precision or minimum detectable change, and power, if appropriate. These parameters can then be considered when determining treatment success either by comparison to quantitative objectives or when comparing treatment to control data.
Confidence intervals are the intervals surrounding a sample mean, that we know with a level of certainty, contain the value of the true parameter. Confidence intervals are very useful for graphical analysis of treatment success. Comparing means and confidence intervals of control and treatment plots side by side or examining the confidence interval of the difference are useful methods of viewing monitoring data. Overlapping confidence intervals often indicate two means cannot be proven to be different, but the confidence intervals also need to be evaluated. For instance, very wide confidence intervals - one that is greater than 50 percent of the mean - may not be considered adequate to perform any comparisons. Often, additional sampling would be needed to decrease the width of the confidence intervals.
The number of samples required to estimate a parameter to a desired level of precision - confidence interval half-width - or to detect a certain magnitude of change -minimum detectable change, or MDC - can also be used to assess data quality. This number can be estimated using sample-size equations which use the standard deviation of an initial sample or known variance to estimate the number of samples required to achieve a desired precison or MDC. There are several different sample size equations depending on which comparison you will be performing. The number of plots required will depend on the variability of the plant community and the type of analysis you are performing. While the number of plots required will vary depending on the location, three plots are needed to generate an estimate of variability and should be considered an absolute minimum number of both control and treatment plots for any monitoring unit. Five plots are usually better.
Power is an estimate of the chance you have made a type II error, or concluding there was no change when there actually was a change. Power is applicable when comparing treatment plots to control or reference plots, but not when comparing treatment plots to a defined standard.
Knowing the level of data quality attained will aid in using limited information to determine treatment success. In general, attaining high data quality on a landscape scale is difficult. For example, sample-size requirements are easier to obtain in plant communities that are uniform and difficult to achieve in communities that are highly variable. Additionally, sample-size requirements will rarely be achieved at the species level when monitoring large post-fire treatment areas. Because of this, it is useful to estimate sample size based on life form. For example, sample size requirements calculated for all perennial seeded grasses will be lower than for each species of perennial seeded grass individually. Additionally, the intensity of sampling and resulting data quality will often be limited by budget and time constraints.
Statistical Analysis
Use of the five common elements - objectives, stratification, control plots, random sampling, and data quality - will facilitate evaluation of the success of post-fire treatments in a defensible statistical analysis. The simplest forms of analysis are to graphically compare treatment plots with control plots or to treatment plots to quantitative objectives. Alternatively, treatment data can be compared to a control or reference plot using a t-test. There are many statistical software packages that will perform these calculations, or they can be accomplished by hand. At the project level this will result in a determination of treatment success for a monitoring unit or project. An MS Excel spreadsheet has been created to help with statistical analysis and sample size estimation and can be requested by sending an e-mail to fresc_outreach@usgs.gov.
Additional Resources:
- The Jornada, Rangeland Research Programs, Database for Inventory, Monitoring & Assessment (DIMA)
- Quantitative Techniques Dataforms
- What types of elevation datasets are available, what formats do they come in, and where can I download them?
- Shapefiles for soil mapping units
- Field book for Describing and Sampling Soils
- Web Soil Survey
- Official Soil Series Descriptions
- Ecological Site Descriptions
- USGS Status and Trends Program
- Measuring Erosion with Silt Fences
Information on this site refers to:
Wirth, T.A., Pyke, D.A., 2007, Monitoring Post-fire Vegetation Rehabilitation Projects- A Common Approach for Non-forested Ecosystems (ES&R): U.S. Geological Survey Scientific Investigations Report 2006-5048, p. 36.
Below are other science projects related to this effort.
Restoration and Ecology of Arid Lands Team (FRESC)
Fire Rehabilitation Effects and Effectiveness
Fire rehabilitation programs have existed within federal agencies since the early 1960s. The U.S. Forest Service and Bureau of Land Management are the largest users of emergency stabilization and rehabilitation (ES&R) funds, but these agencies only sporadically implement proposed monitoring and rarely use common protocols. As a result, it is impossible to draw scientifically credible conclusions regarding the effectiveness of ES&R projects. Additionally, the data that does exist are stored in field office files and are often not available for other managers to use when planning new ES&R treatments.
A common monitoring strategy helps address concerns through
- Implementation of a common approach and methodology to assess the effectiveness of ES&R projects region-wide
- Use of field based computers to enter data in a standard format that is subequently uploaded to a centralized database
- Analysis of collected data at regular intervals to determine overall success and to identify factors involved in successful treatments
By following this strategy, managers can ultimately determine the effectiveness of post-fire rehabilitation activities region-wide.
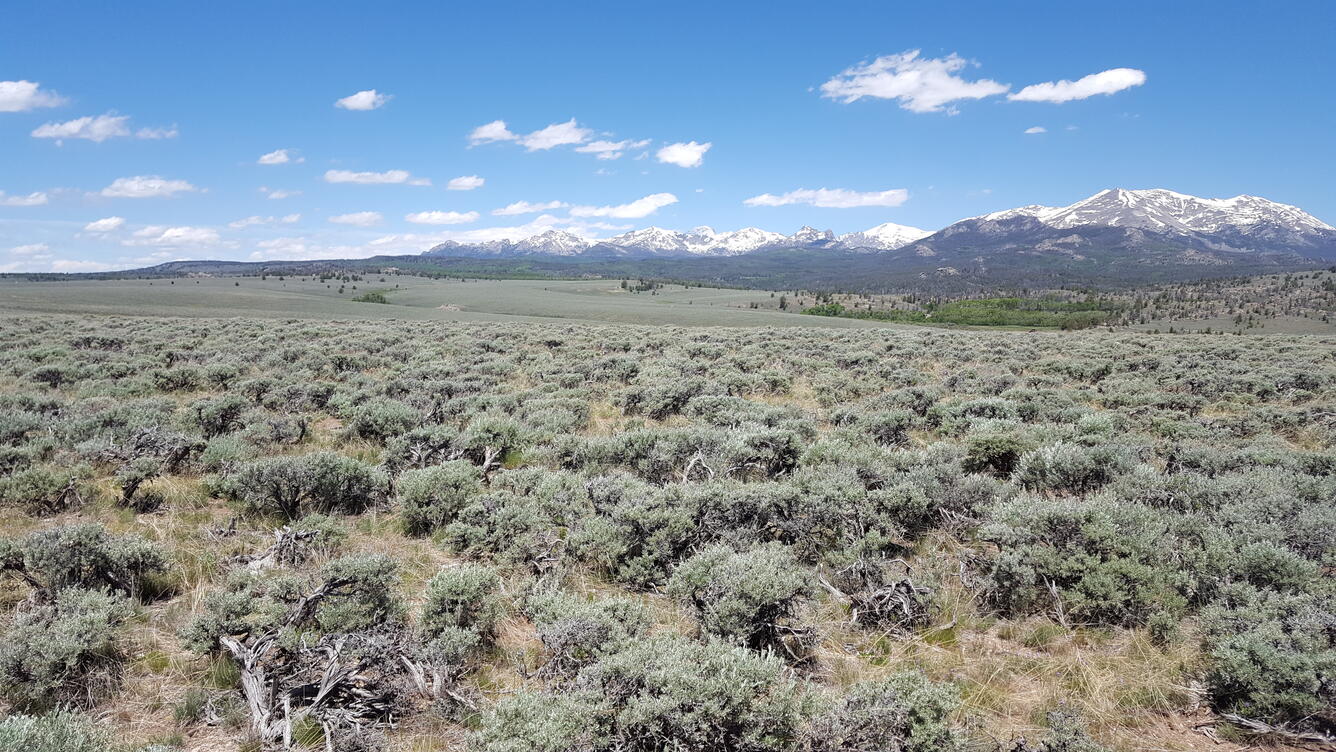
The Approach
We reviewed seven vegetation monitoring manuals produced by federal agencies to determine which elements would be appropriate for evaluating ES&R treatment success.
Monitoring Manual for Grasslands, Shrublands, and Savanna Ecosystems (Volumes 1 and 2)
Herrick, J.E., Van Zee, J.W., Havstad, K.M., Burkett, L.M., Whitford, W.G. 2005a. Monitoring Manual For Grassland, Shrubland and Savanna Ecosystems. Volume 1: Quick Start. USDA-ARS Jornada Experimental Range. 36 p.
Herrick, J.E., Van Zee, J.W., Havstad, K.M, Burkett, L.M., Whitford, W.G. 2005b. Monitoring Manual For Grassland, Shrubland and Savanna Ecosystems. Volume 2: Design, Supplementary Methods and Interpretation. USDA-ARS Jornada Experimental Range. 200 p.
Measuring and monitoring plant populations
Elzinga, C.L., Salzer, D.W., and Willoughby, J.W. 1998. Measuring and Monitoring Plant Populations. USDI Bureau of Land Management Technical Reference 1730-1. 492p.
Sampling vegetation attributes
Interagency Technical Reference. 1999. Sampling Vegetation Attributes. BLM Technical Reference 1734-4. 158 p.
Fire Effects Monitoring and Inventory Protocol (FIREMON)
Lutes, Duncan C., Keane, Robert, E., Caratti, John. F., Key, Carl H., Benson, Nathan C., Sutherland, Steve, Gangi, Larry J., 2006. FIREMON: Fire Effects Monitoring and Inventory System. Gen. Tech. Rep. RMRS-GTR-164-CD. For Collins, CO: U.S. Department of Agriculture, Forest Service, Rocky Mountain Research Station. 1 CD. 400 p.
USDI National Park Service. 2003. Fire Monitoring Handbook. Boise, ID: Fire Management program Center, National Interagency Fire Center. 274 p.
Fuel and Fire Effects Monitoring Guide
USDI Fish and Wildlife Service. 1999. Fuel and Fire Effects Monitoring Guide.
Land Condition Trend Analysis
U.S. Army Sustainable Range Program, 2006. RTLA Technical Reference Manual: Ecological Monitoring on Military Lands.
The following are descriptions of program design elements found within the reviewed monitoring manuals that are suitable for use in evaluating ES&R treatment success.
Objectives
All seven manuals included objectives as an important element of a monitoring program. Several manuals also provide good descriptions of how to formulate objectives.
Measuring and Monitoring Plant Populations inidcates both management and sampling objectives should be written for any project. Management objectives are “clearly articulated descriptions of a measurable standard, desired state, threshold value, amount of change, or trend that you are striving to achieve for a particular plant population or habitat characteristics.”
Well-defined management objectives in a monitoring program perform two functions.
- They establish a standard to measure the degree of success, and
- They determine the appropriate indicators to measure.
A standard protocol can then be followed for the measurement of each indicator. Thus, data collection activities are directly related to management objectives. Sampling objectives should be paired with each management objective and specify the desired confidence level, precision - confidence interval width, level of type II error, or detectable change for the sampling effort.
Stratification
Five of the seven monitoring manuals describe stratification. Stratification is the partitioning of treatment areas to reduce variation and increase precision of sampling efforts. Areas that may respond differently to ES&R treatments such as different soil types or ecological sites are good candidates for strata.
Rules for stratification of treatment areas into monitoring units should be created during the planning stage of an ES&R project. Stratification can be undertaken concurrently with or after identification of treatment areas. In many cases, stratification is completed as a by-product of treatment planning such as assigning different seed mixes to sites with different characteristics or potentials.
Background information on the treatment area is essential for stratification. A variety of GIS data are useful for delineating monitoring units, including digital elevation models (DEMs), fire perimeters, proposed and actual treatment areas, soils (if available), roads, and land-use information. Monitoring units can be derived with GIS software, such as ArcGIS, based on the available information and the specifics of the project.
- Shapefiles for ecological sites may be the preferred initial strata, if available.
- If only soils are available, then the site can be divided initially into soil strata separately to reduce variation and increase monitoring efficiency.
- If only soil surveys are available, but soil-to-ecological site correlations are known, then differing soils that correlate to the same ecological site may be combined into the same strata.
- Slope classes can be generated from DEMs when seedings will occur over a large range of slopes.
- Areas that are not likely to be seeded due to topography can be excluded from the monitoring unit using DEMs.
Stratification of the area for both treatments and monitoring can be accomplished using a defined set of variables such as slope, aspect, elevation, treatment type, minimum size, soil type, or ecological site.
Descriptions of monitoring units should be included in monitoring plans so that the scope of inference is known. For example:
- Monitoring unit 1 includes all areas of less than 20 percent slope within soil type A in the native seed mix treatment.
- Monitoring unit 2 includes all areas equal to or greater than 20 percent slope within soil type A in the native seed mix treatment.
- Monitoring unit 3 includes all areas of less than 20 percent slope within the non-native seed mix treatment.
Similar methods of stratification used on different projects will facilitate comparisons among those projects and aid region-wide assessments of ES&R treatment effectiveness. Additional information on stratification can be found in the Fire Monitoring Handbook, Fire Effects Monitoring and Inventory Protocol, and the Monitoring Manual for Grassland, Shrubland, and Savanna Ecosystems.
Control Plots
Control plots are locations within a proposed treatment area that are established prior to and avoided when treatments are implemented. They provide important information on natural recovery that can be used to determine whether or not treatments were necessary in the first place. This is especially useful given limited resources for implementing large projects in severe fire years. The Fire Monitoring Handbook states that control areas should be used when attributing a particular effect to the applied treatment. The goal is to show that the treatment caused the observed change.
Prior to applying treatments, a minimum of three control plots should be randomly placed within each monitoring unit. Control plots should not be placed in adjacent untreated areas because they were not proposed to be treated and are therefore different from those areas that were proposed to be treated. Since adjacent areas are most likely different from the area being treated, they make poor controls.
Control plots may not be practical in all situations such as in cases where life or property are threatened. These situations often occur when the areas to be treated are on slopes above developments and it is not possible to leave some areas untreated. However, controls should be used whenever possible because they provide the best measure of natural regeneration and ES&R treatment success.
Random Sampling
Random sampling ensures that monitoring data are unbiased and representative of the monitoring unit. While this may be time-consuming, it is essential for defensible monitoring data and success determinations. Without random sampling, criticism may include that the samples only came from areas where the treatments were effective, or that data were biased by the site-selection process. In addition, data that are not derived from random sampling are only valid for the plot at which they were collected and cannot be used to infer to the rest of the treatment area.
There are several different methods of random sampling that can be used to monitor ES&R treatments: simple, systematic, restricted, or two-stage random sampling. One of these methods should be used to enable statistical inference over as much of the treated area as possible. To generate random samples, there are several options, including grids placed over maps or GIS random-point generators.
Rejection criteria should be defined and procedures established if random plot locations occur in areas that cannot be seeded, such as roads, rocky outcrops, steep slopes, streams, or other features. In some cases it may be possible to relocate the plot nearby in a random direction. Or, it may be necessary to move to the next randomly generated point.
Data Quality
After collecting monitoring data, it is helpful to determine how well it can assess ES&R treatment success. Parameters that should be examined are confidence intervals, sample-size estimates, precision or minimum detectable change, and power, if appropriate. These parameters can then be considered when determining treatment success either by comparison to quantitative objectives or when comparing treatment to control data.
Confidence intervals are the intervals surrounding a sample mean, that we know with a level of certainty, contain the value of the true parameter. Confidence intervals are very useful for graphical analysis of treatment success. Comparing means and confidence intervals of control and treatment plots side by side or examining the confidence interval of the difference are useful methods of viewing monitoring data. Overlapping confidence intervals often indicate two means cannot be proven to be different, but the confidence intervals also need to be evaluated. For instance, very wide confidence intervals - one that is greater than 50 percent of the mean - may not be considered adequate to perform any comparisons. Often, additional sampling would be needed to decrease the width of the confidence intervals.
The number of samples required to estimate a parameter to a desired level of precision - confidence interval half-width - or to detect a certain magnitude of change -minimum detectable change, or MDC - can also be used to assess data quality. This number can be estimated using sample-size equations which use the standard deviation of an initial sample or known variance to estimate the number of samples required to achieve a desired precison or MDC. There are several different sample size equations depending on which comparison you will be performing. The number of plots required will depend on the variability of the plant community and the type of analysis you are performing. While the number of plots required will vary depending on the location, three plots are needed to generate an estimate of variability and should be considered an absolute minimum number of both control and treatment plots for any monitoring unit. Five plots are usually better.
Power is an estimate of the chance you have made a type II error, or concluding there was no change when there actually was a change. Power is applicable when comparing treatment plots to control or reference plots, but not when comparing treatment plots to a defined standard.
Knowing the level of data quality attained will aid in using limited information to determine treatment success. In general, attaining high data quality on a landscape scale is difficult. For example, sample-size requirements are easier to obtain in plant communities that are uniform and difficult to achieve in communities that are highly variable. Additionally, sample-size requirements will rarely be achieved at the species level when monitoring large post-fire treatment areas. Because of this, it is useful to estimate sample size based on life form. For example, sample size requirements calculated for all perennial seeded grasses will be lower than for each species of perennial seeded grass individually. Additionally, the intensity of sampling and resulting data quality will often be limited by budget and time constraints.
Statistical Analysis
Use of the five common elements - objectives, stratification, control plots, random sampling, and data quality - will facilitate evaluation of the success of post-fire treatments in a defensible statistical analysis. The simplest forms of analysis are to graphically compare treatment plots with control plots or to treatment plots to quantitative objectives. Alternatively, treatment data can be compared to a control or reference plot using a t-test. There are many statistical software packages that will perform these calculations, or they can be accomplished by hand. At the project level this will result in a determination of treatment success for a monitoring unit or project. An MS Excel spreadsheet has been created to help with statistical analysis and sample size estimation and can be requested by sending an e-mail to fresc_outreach@usgs.gov.
Additional Resources:
- The Jornada, Rangeland Research Programs, Database for Inventory, Monitoring & Assessment (DIMA)
- Quantitative Techniques Dataforms
- What types of elevation datasets are available, what formats do they come in, and where can I download them?
- Shapefiles for soil mapping units
- Field book for Describing and Sampling Soils
- Web Soil Survey
- Official Soil Series Descriptions
- Ecological Site Descriptions
- USGS Status and Trends Program
- Measuring Erosion with Silt Fences
Information on this site refers to:
Wirth, T.A., Pyke, D.A., 2007, Monitoring Post-fire Vegetation Rehabilitation Projects- A Common Approach for Non-forested Ecosystems (ES&R): U.S. Geological Survey Scientific Investigations Report 2006-5048, p. 36.
Below are other science projects related to this effort.