dbSEABED: Data processing and mining
At the core of usSEABED is dbSEABED, a data-mining program based on the application of fuzzy set theory to marine geological and biological data. Fuzzy set theory allows expansion of coverage of the seafloor by the use of word-based data from core logs, sample descriptions, photos, and videos, as well as the more standard numeric data from a laboratory.
The dbSEABED program, in part, parses word-based descriptive data such as "brown fine sand with abundant shells; seagrass and some pebbles; whiff of h2s" into numeric, georeferenced data. This process extends the data coverage of the seabed by using words, an important data type characterizing the seabed. While a simplified explanation of the parsing process is provided, more information can be found within the usSEABED publications.
The dbSEABED program applies fuzzy set theory concepts to geological descriptions, using:
- a parser that divides the descriptions into arithmetic equations;
- a thesaurus that attaches meanings and memberships to the quantifiers, modifiers, and objects; and
- a linear weighted assembly of the numerical totals.
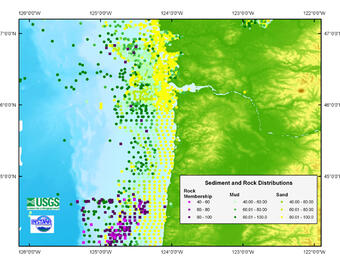
In the dbSEABED program, word memberships can be defined across many parameters—not just grain size. The outputs are numeric values, representing fuzzy memberships of parameters such as mud, grain sizes, carbonate, organic carbon, grain types, sedimentary features, rock, weed coverages, and engineering strengths.
Users of the usSEABED dataset based on descriptive data should be aware of the nature of the data; that is, fuzzy memberships that are best thought of as a measure of truth or possibility, neither probability nor the results of rigorous analytical methods.
Ongoing statistical comparisons are made between the lab-based and word-based data outputs for calibration of the parsing process, with a goal of no more than one phi size between the parsed and lab-based outputs from the same sample. Larger differences in some samples may be due to an inherent difference in the sample analyzed: for instance, the parsed output may be on the whole sample including stones, shells, or other large objects, and the lab-based data may be from the analysis of the matrix only. Each user may choose which output type (or both) fit the needs of a given study.
Learn more about usSEABED.
usSEABED
Accessing usSEABED
usSEABED data format and content
dbSEABED: Data processing and mining
Parsing in dbSEABED
At the core of usSEABED is dbSEABED, a data-mining program based on the application of fuzzy set theory to marine geological and biological data. Fuzzy set theory allows expansion of coverage of the seafloor by the use of word-based data from core logs, sample descriptions, photos, and videos, as well as the more standard numeric data from a laboratory.
The dbSEABED program, in part, parses word-based descriptive data such as "brown fine sand with abundant shells; seagrass and some pebbles; whiff of h2s" into numeric, georeferenced data. This process extends the data coverage of the seabed by using words, an important data type characterizing the seabed. While a simplified explanation of the parsing process is provided, more information can be found within the usSEABED publications.
The dbSEABED program applies fuzzy set theory concepts to geological descriptions, using:
- a parser that divides the descriptions into arithmetic equations;
- a thesaurus that attaches meanings and memberships to the quantifiers, modifiers, and objects; and
- a linear weighted assembly of the numerical totals.
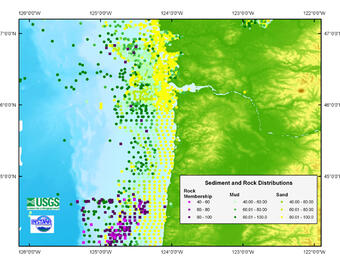
In the dbSEABED program, word memberships can be defined across many parameters—not just grain size. The outputs are numeric values, representing fuzzy memberships of parameters such as mud, grain sizes, carbonate, organic carbon, grain types, sedimentary features, rock, weed coverages, and engineering strengths.
Users of the usSEABED dataset based on descriptive data should be aware of the nature of the data; that is, fuzzy memberships that are best thought of as a measure of truth or possibility, neither probability nor the results of rigorous analytical methods.
Ongoing statistical comparisons are made between the lab-based and word-based data outputs for calibration of the parsing process, with a goal of no more than one phi size between the parsed and lab-based outputs from the same sample. Larger differences in some samples may be due to an inherent difference in the sample analyzed: for instance, the parsed output may be on the whole sample including stones, shells, or other large objects, and the lab-based data may be from the analysis of the matrix only. Each user may choose which output type (or both) fit the needs of a given study.
Learn more about usSEABED.