This a version of the logo for the Python Hyperspectral Analysis Tool (PyHAT). It is intended for use in info boxes on the USGS website. The spectrum in the graphic is a laser induced breakdown spectroscopy spectrum, plotted on a logarithmic y axis to emphasize weaker emission peaks.
Ryan Bradley Anderson
Ryan Anderson is a planetary scientist at the U.S. Geological Survey Astrogeology Science Center and science team member on the Curiosity and Perseverance Mars rover missions.
Ryan attended the University of Michigan, where he double majored in Physics and Astronomy, and Cornell University where received his PhD in Planetary Science. He conducted a detailed study of Gale crater, the landing site for the Mars Science Laboratory Rover (MSL), and is a member of the ChemCam instrument science team. Ryan is also a member of the science team on the SuperCam instrument on the Perseverance rover. His work with ChemCam and SuperCam focuses on improving the accuracy of the chemistry measurements made by the instruments. In addition to his research and mission operations, Ryan is passionate about communicating science to the public, and serves as Communication Lead for the Astrogeology Science Center. He enjoys teaching and public speaking and for several years ran a planetary science-themed blog called The Martian Chronicles, which was one of the founding blogs of the American Geophysical Union blogosphere. That blog evolved into a group blog hosted by NASA JPL that shares updates on what the Curiosity rover is currently doing.
Professional Experience
February 2021 - Present: Perseverance Mars rover operations
September 2014 - Present: Physical Scientist, USGS Astrogeology Science Center
July 2014 - Present: SuperCam science team member (Perseverance Mars rover)
August 2012 - Present: Curiosity Mars rover operations
January 2012 - Present: ChemCam science team member (Curiosity Mars rover)
January 2012 - September 2014: Shoemaker Postdoctoral Fellow, USGS Astrogeology Science Center
2007 - 2014: Mars Exploration Rover (MER) mission operations (Pancam Payload Downlink Lead)
Education and Certifications
PhD, Astronomy (Geology minor), Cornell University, 2012
B.S., Astronomy and Astrophysics, University of Michigan, 2006
B.S., Physics, University of Michigan, 2006
Science and Products
This a version of the logo for the Python Hyperspectral Analysis Tool (PyHAT). It is intended for use in info boxes on the USGS website. The spectrum in the graphic is a laser induced breakdown spectroscopy spectrum, plotted on a logarithmic y axis to emphasize weaker emission peaks.
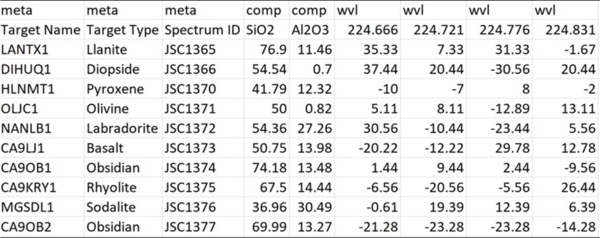
Screenshot showing the simple data format used by the Python Hyperspectral Analysis Tool (PyHAT). Spectra are stored in rows of the table, along with their associated metadata and compositional information.
Screenshot showing the simple data format used by the Python Hyperspectral Analysis Tool (PyHAT). Spectra are stored in rows of the table, along with their associated metadata and compositional information.
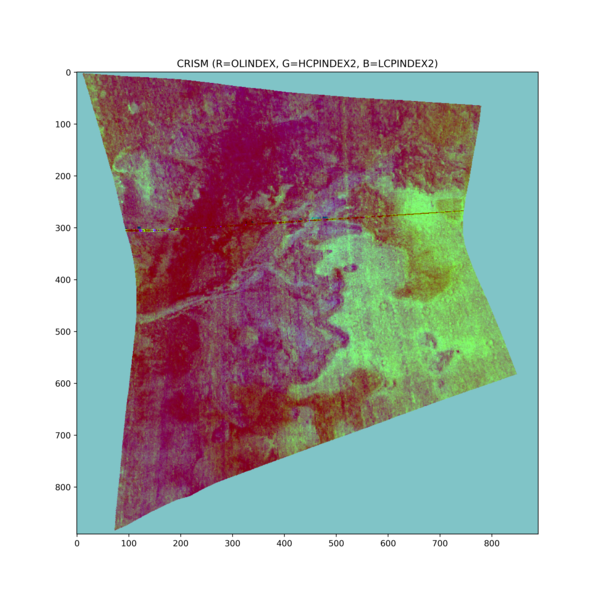
This figure shows an example mineral parameter map image generated using PyHAT. The area in this Compact Reconnaissance Imaging Spectrometer for Mars (CRISM) image is Jezero crater, the landing site of NASA's Mars Perseverance rover.
This figure shows an example mineral parameter map image generated using PyHAT. The area in this Compact Reconnaissance Imaging Spectrometer for Mars (CRISM) image is Jezero crater, the landing site of NASA's Mars Perseverance rover.
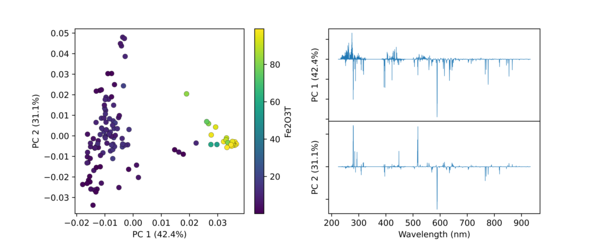
This figure shows an example PCA plot generated using PyHAT. The input data were laser induced breakdown spectroscopy (LIBS) spectra. PyHAT was used to apply a baseline correction and normalization to the total intensity for each spectrum.
This figure shows an example PCA plot generated using PyHAT. The input data were laser induced breakdown spectroscopy (LIBS) spectra. PyHAT was used to apply a baseline correction and normalization to the total intensity for each spectrum.
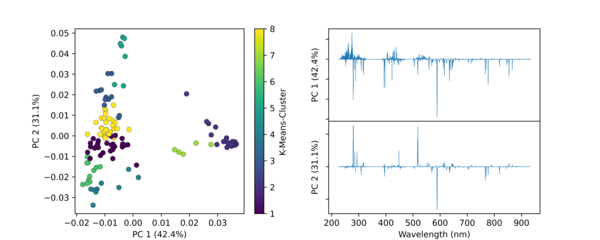
Python Hyperspectral Analysis Tool (PyHAT) Principal Component Analysis K-Means Clustering Example
linkThis figure shows an example PCA plot generated using PyHAT. The input data were laser induced breakdown spectroscopy (LIBS) spectra. PyHAT was used to apply a baseline correction and normalization to the total intensity for each spectrum.
Python Hyperspectral Analysis Tool (PyHAT) Principal Component Analysis K-Means Clustering Example
linkThis figure shows an example PCA plot generated using PyHAT. The input data were laser induced breakdown spectroscopy (LIBS) spectra. PyHAT was used to apply a baseline correction and normalization to the total intensity for each spectrum.
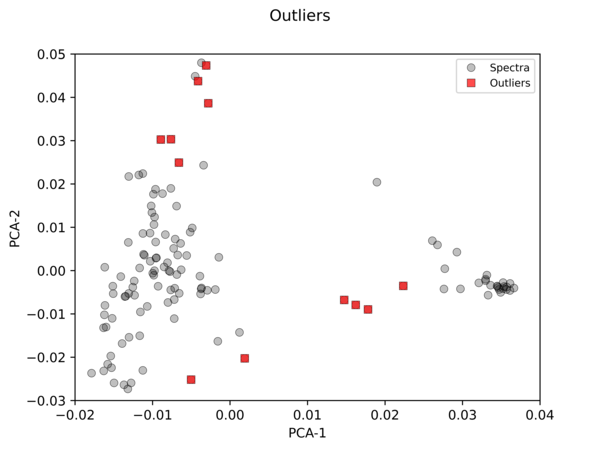
This figure shows an example of outlier identification using PyHAT. The input data were laser induced breakdown spectroscopy (LIBS) spectra. PyHAT was used to apply a baseline correction and normalization to the total intensity for each spectrum. Dimensionality was then reduced using principal components analysis (PCA).
This figure shows an example of outlier identification using PyHAT. The input data were laser induced breakdown spectroscopy (LIBS) spectra. PyHAT was used to apply a baseline correction and normalization to the total intensity for each spectrum. Dimensionality was then reduced using principal components analysis (PCA).
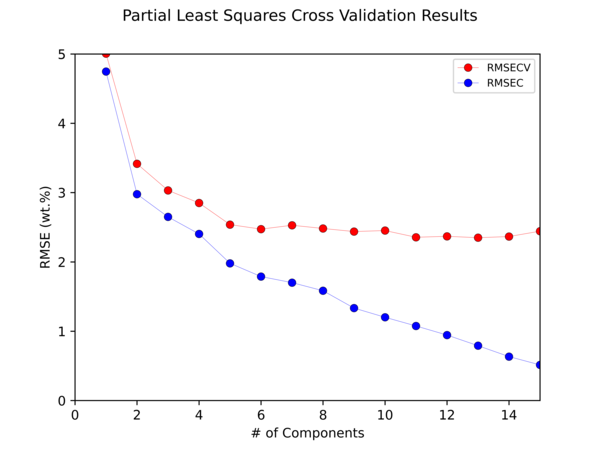
This figure shows the results of cross-validating a Partial Least Squares (PLS) model to predict the abundance of CaO in geologic targets using PyHAT. Cross validation is necessary to optimize the parameters of a regression algorithm to avoid overfitting.
This figure shows the results of cross-validating a Partial Least Squares (PLS) model to predict the abundance of CaO in geologic targets using PyHAT. Cross validation is necessary to optimize the parameters of a regression algorithm to avoid overfitting.
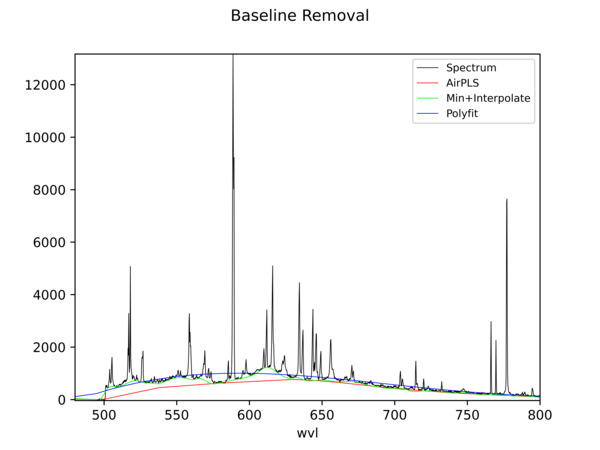
This figure shows an example spectrum plot generated using PyHAT. The black line is a laser induced breakdown spectroscopy (LIBS) spectrum of a basalt sample. The colored lines show the baseline estimated using several different algorithms.
This figure shows an example spectrum plot generated using PyHAT. The black line is a laser induced breakdown spectroscopy (LIBS) spectrum of a basalt sample. The colored lines show the baseline estimated using several different algorithms.
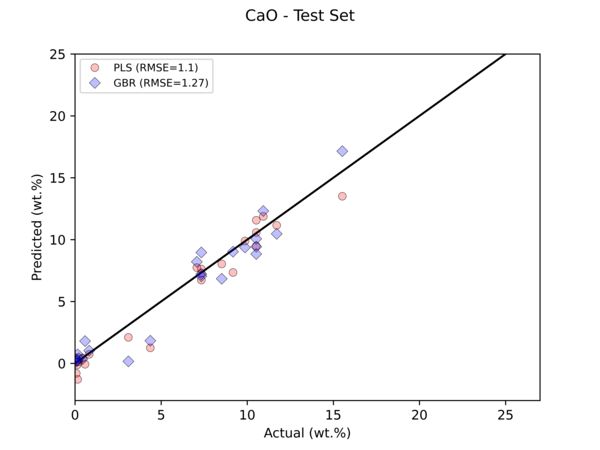
This figure compares the results of two regression models to predict the abundance of CaO in geologic standards based on their laser induced breakdown spectroscopy (LIBS) spectra using PyHAT. The horizontal axis is the independently measured CaO abundance, the vertical axis is the abundance predicted by the models.
This figure compares the results of two regression models to predict the abundance of CaO in geologic standards based on their laser induced breakdown spectroscopy (LIBS) spectra using PyHAT. The horizontal axis is the independently measured CaO abundance, the vertical axis is the abundance predicted by the models.
Regolith of the crater floor units, Jezero crater, Mars: Textures, composition and implications for provenance
Spatial and temporal distribution of sinuous ridges in southeastern Terra Sabaea and the northern region of Hellas Planitia, Mars
Morphology and paleohydrology of intracrater alluvial fans north of Hellas Basin, Mars
Overview of the morphology and chemistry of diagenetic features in the clay-rich Glen Torridon Unit of Gale Crater, Mars
Introduction to the Python Hyperspectral Analysis Tool (PyHAT)
Post-landing major element quantification using SuperCam laser induced breakdown spectroscopy
Improving ChemCam LIBS long-distance elemental compositions using empirical abundance trends
Quantification of manganese for ChemCam Mars and laboratory spectra using a multivariate model
Alternating wet and dry depositional environments recorded in the stratigraphy of Mt Sharp at Gale Crater, Mars
Laser-induced breakdown spectroscopy
The mineral diversity of Jezero crater: Evidence for possible lacustrine carbonates on Mars
Complex bedding geometry in the upper portion of Aeolis Mons, Gale crater, Mars
Python Hyperspectral Analysis Tool (PyHAT)
Science and Products
This a version of the logo for the Python Hyperspectral Analysis Tool (PyHAT). It is intended for use in info boxes on the USGS website. The spectrum in the graphic is a laser induced breakdown spectroscopy spectrum, plotted on a logarithmic y axis to emphasize weaker emission peaks.
This a version of the logo for the Python Hyperspectral Analysis Tool (PyHAT). It is intended for use in info boxes on the USGS website. The spectrum in the graphic is a laser induced breakdown spectroscopy spectrum, plotted on a logarithmic y axis to emphasize weaker emission peaks.
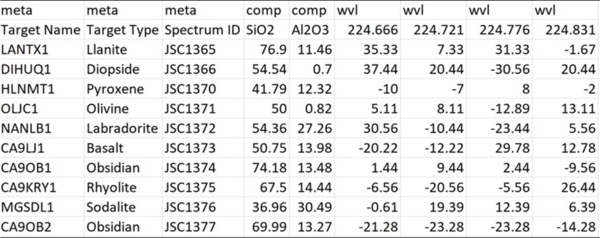
Screenshot showing the simple data format used by the Python Hyperspectral Analysis Tool (PyHAT). Spectra are stored in rows of the table, along with their associated metadata and compositional information.
Screenshot showing the simple data format used by the Python Hyperspectral Analysis Tool (PyHAT). Spectra are stored in rows of the table, along with their associated metadata and compositional information.
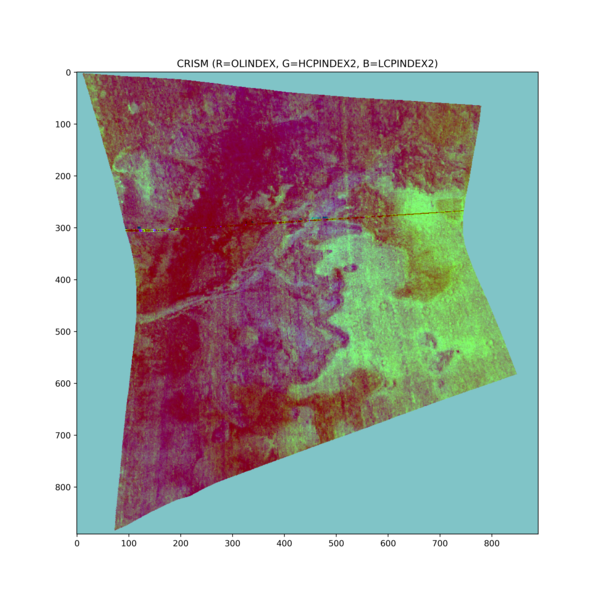
This figure shows an example mineral parameter map image generated using PyHAT. The area in this Compact Reconnaissance Imaging Spectrometer for Mars (CRISM) image is Jezero crater, the landing site of NASA's Mars Perseverance rover.
This figure shows an example mineral parameter map image generated using PyHAT. The area in this Compact Reconnaissance Imaging Spectrometer for Mars (CRISM) image is Jezero crater, the landing site of NASA's Mars Perseverance rover.
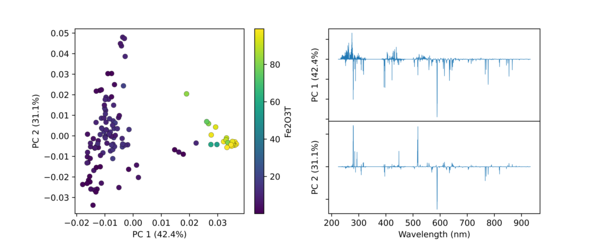
This figure shows an example PCA plot generated using PyHAT. The input data were laser induced breakdown spectroscopy (LIBS) spectra. PyHAT was used to apply a baseline correction and normalization to the total intensity for each spectrum.
This figure shows an example PCA plot generated using PyHAT. The input data were laser induced breakdown spectroscopy (LIBS) spectra. PyHAT was used to apply a baseline correction and normalization to the total intensity for each spectrum.
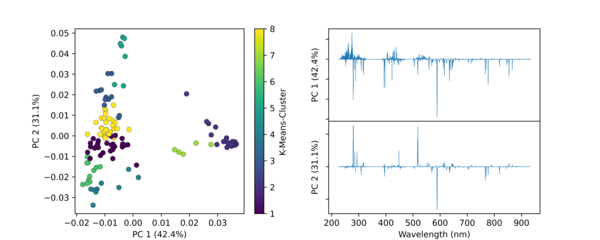
Python Hyperspectral Analysis Tool (PyHAT) Principal Component Analysis K-Means Clustering Example
linkThis figure shows an example PCA plot generated using PyHAT. The input data were laser induced breakdown spectroscopy (LIBS) spectra. PyHAT was used to apply a baseline correction and normalization to the total intensity for each spectrum.
Python Hyperspectral Analysis Tool (PyHAT) Principal Component Analysis K-Means Clustering Example
linkThis figure shows an example PCA plot generated using PyHAT. The input data were laser induced breakdown spectroscopy (LIBS) spectra. PyHAT was used to apply a baseline correction and normalization to the total intensity for each spectrum.
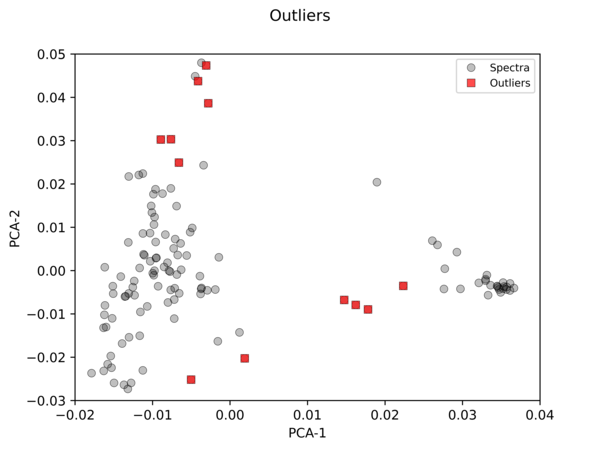
This figure shows an example of outlier identification using PyHAT. The input data were laser induced breakdown spectroscopy (LIBS) spectra. PyHAT was used to apply a baseline correction and normalization to the total intensity for each spectrum. Dimensionality was then reduced using principal components analysis (PCA).
This figure shows an example of outlier identification using PyHAT. The input data were laser induced breakdown spectroscopy (LIBS) spectra. PyHAT was used to apply a baseline correction and normalization to the total intensity for each spectrum. Dimensionality was then reduced using principal components analysis (PCA).
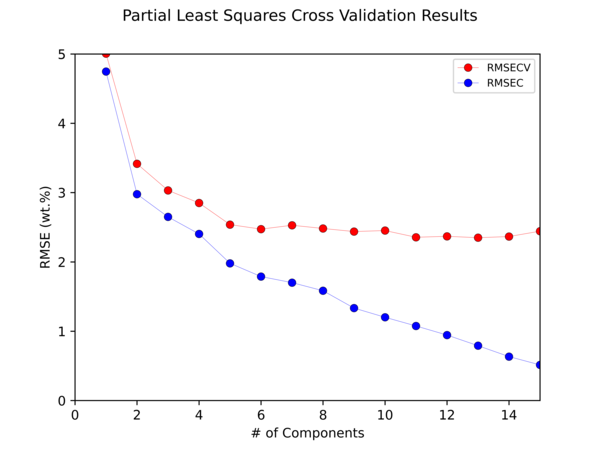
This figure shows the results of cross-validating a Partial Least Squares (PLS) model to predict the abundance of CaO in geologic targets using PyHAT. Cross validation is necessary to optimize the parameters of a regression algorithm to avoid overfitting.
This figure shows the results of cross-validating a Partial Least Squares (PLS) model to predict the abundance of CaO in geologic targets using PyHAT. Cross validation is necessary to optimize the parameters of a regression algorithm to avoid overfitting.
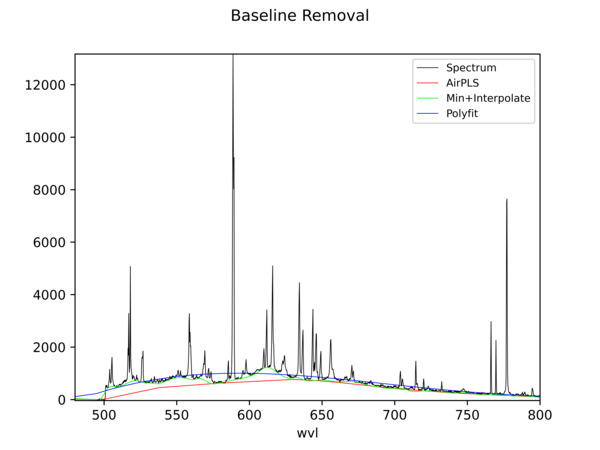
This figure shows an example spectrum plot generated using PyHAT. The black line is a laser induced breakdown spectroscopy (LIBS) spectrum of a basalt sample. The colored lines show the baseline estimated using several different algorithms.
This figure shows an example spectrum plot generated using PyHAT. The black line is a laser induced breakdown spectroscopy (LIBS) spectrum of a basalt sample. The colored lines show the baseline estimated using several different algorithms.
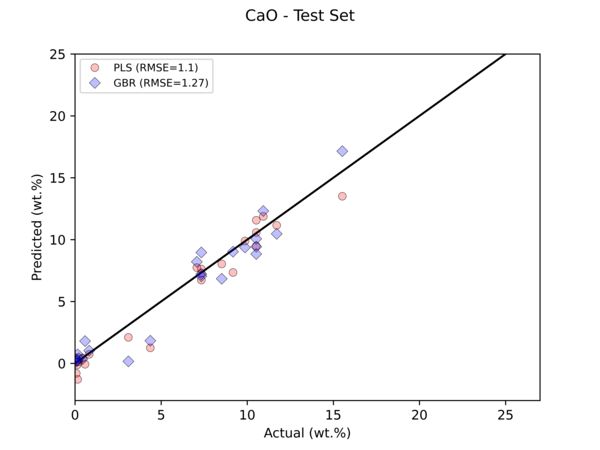
This figure compares the results of two regression models to predict the abundance of CaO in geologic standards based on their laser induced breakdown spectroscopy (LIBS) spectra using PyHAT. The horizontal axis is the independently measured CaO abundance, the vertical axis is the abundance predicted by the models.
This figure compares the results of two regression models to predict the abundance of CaO in geologic standards based on their laser induced breakdown spectroscopy (LIBS) spectra using PyHAT. The horizontal axis is the independently measured CaO abundance, the vertical axis is the abundance predicted by the models.