The US Interagency Elevation Inventory, or USIEI, is a nationwide listing of known publicly available high-accuracy topographic and bathymetric source elevation data for the United States and its territories. The inventory provides a single resource for information about all known completed and in-progress broad-area public domain elevation data.
Lesson 10c2: Exploring and Classifying LiDAR Data in Global Mapper
Detailed Description
This lesson will cover additional LiDAR point cloud processing and analysis features in Global Mapper.
Please note that some of our URLs in the video have changed. All URLs beginning with 'viewer.nationalmap.gov/...' have been updated to 'apps.nationalmap.gov/...'. These URLs should reroute automatically, but if you have an issue replace 'viewer.nationalmap.gov/...' with 'apps.nationalmap.gov/...'. If you have questions, please visit https://usgs.gov/NationalMap/data
This lesson contains links to data hosted at an ftp site which was active when this video was published. That site is no longer functional. Please substitute the following address in place of the ftp address: https://rockyweb.usgs.gov/Training_Data/
Details
Sources/Usage
Public Domain.
Related Content
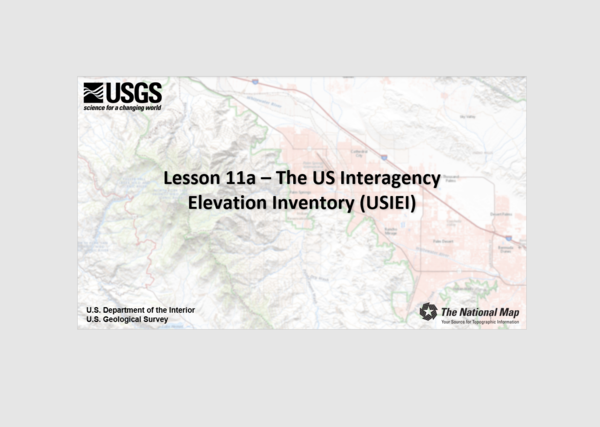
The US Interagency Elevation Inventory, or USIEI, is a nationwide listing of known publicly available high-accuracy topographic and bathymetric source elevation data for the United States and its territories. The inventory provides a single resource for information about all known completed and in-progress broad-area public domain elevation data.
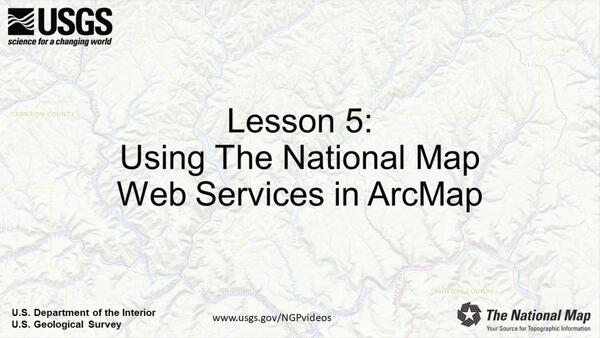
In this lesson, we will provide an overview and demonstrate how to use The National Map’s web services in a mapping application. Although downloading data from The National Map is a popular option for many users, there are numerous situations where it may be preferable to simply call TNM web services into your mapping application.
In this lesson, we will provide an overview and demonstrate how to use The National Map’s web services in a mapping application. Although downloading data from The National Map is a popular option for many users, there are numerous situations where it may be preferable to simply call TNM web services into your mapping application.
This lesson will cover Stream Flow Estimates in NHDPlus High Resolution. Enhanced Runoff Method, or EROM is the method used to compute estimates of the mean annual flow for the NHDPlus flowline features in the NHDPlus HR network.
This lesson will cover Stream Flow Estimates in NHDPlus High Resolution. Enhanced Runoff Method, or EROM is the method used to compute estimates of the mean annual flow for the NHDPlus flowline features in the NHDPlus HR network.
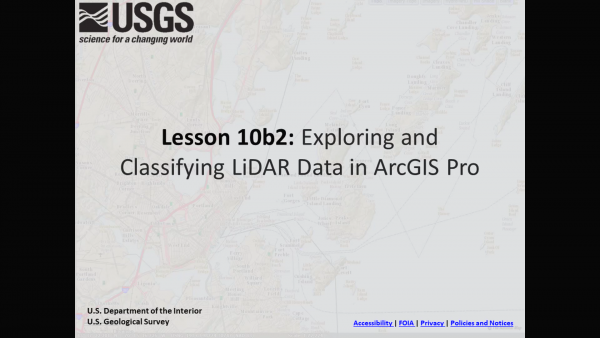
This lesson will cover additional lidar point cloud processing and analysis features in ArcGIS Pro. If necessary, please review lesson 10b1 for guidance with importing LAS files, filtering, and visualizing lidar point cloud data in ArcGIS Pro.
This lesson will cover additional lidar point cloud processing and analysis features in ArcGIS Pro. If necessary, please review lesson 10b1 for guidance with importing LAS files, filtering, and visualizing lidar point cloud data in ArcGIS Pro.
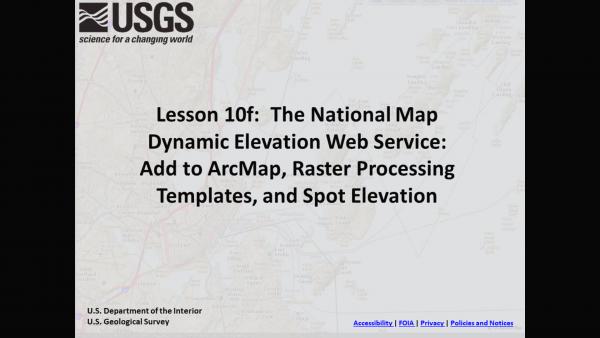
This video tutorial shows how to use the National Map’s 3D Elevation Program (3DEP) Elevation web service in ArcMap. By the end of this lesson, you will be able to add the Dynamic Elevation Web Coverage Service to ArcMap, enable raster processing templates, and retrieve a spot elevation.
This video tutorial shows how to use the National Map’s 3D Elevation Program (3DEP) Elevation web service in ArcMap. By the end of this lesson, you will be able to add the Dynamic Elevation Web Coverage Service to ArcMap, enable raster processing templates, and retrieve a spot elevation.
In this lesson, you will learn about the lidar products available through the 3D Elevation Program (which is abbreviated as “3DEP”). The lesson will introduce 3DEP, discuss the lidar data available, and where to find the data.
In this lesson, you will learn about the lidar products available through the 3D Elevation Program (which is abbreviated as “3DEP”). The lesson will introduce 3DEP, discuss the lidar data available, and where to find the data.
In this lesson we will examine a brief history of the USGS topographic map, Introduce US Topo maps and their content, discuss the production and future goals of US Topo, and learn how to access these maps for viewing or printing.
In this lesson we will examine a brief history of the USGS topographic map, Introduce US Topo maps and their content, discuss the production and future goals of US Topo, and learn how to access these maps for viewing or printing.
By the end of this lesson, you will understand the difference between the LAS and LAZ format, know where to download the laszip.exe tool, and how to decompress a LAZ file to a LAS file using laszip.exe. The USGS offers other lessons on using LAS files in ArcGIS Pro, Global Mapper, and LP360.
By the end of this lesson, you will understand the difference between the LAS and LAZ format, know where to download the laszip.exe tool, and how to decompress a LAZ file to a LAS file using laszip.exe. The USGS offers other lessons on using LAS files in ArcGIS Pro, Global Mapper, and LP360.
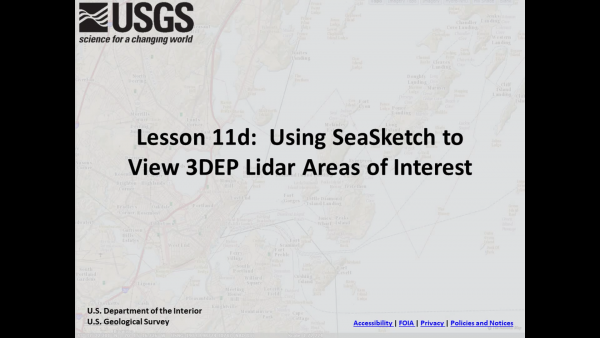
In this lesson you will learn how to zoom to your area of interest and view the footprints of existing and proposed topographic lidar projects.
In this lesson you will learn how to zoom to your area of interest and view the footprints of existing and proposed topographic lidar projects.
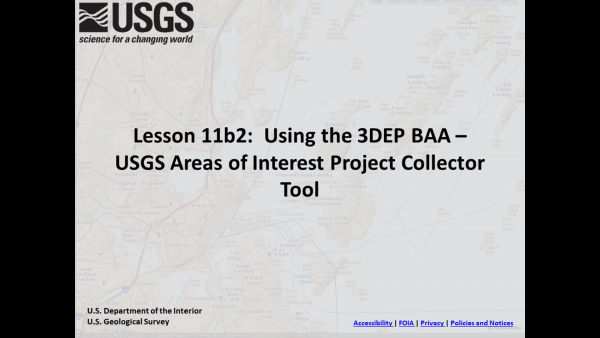
In this lesson you will learn how to use the USGS areas of interest project collection tool to add your lidar project to the Seasketch Demonstration Site for Federal Mapping Data Acquisition Coordination.
In this lesson you will learn how to use the USGS areas of interest project collection tool to add your lidar project to the Seasketch Demonstration Site for Federal Mapping Data Acquisition Coordination.
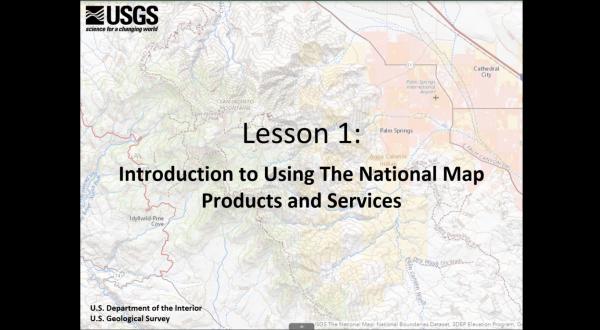
Welcome to the USGS course on Using The National Map Products and Services. This series of videos is focused on The National Map and the variety of products and services that it provides.
Welcome to the USGS course on Using The National Map Products and Services. This series of videos is focused on The National Map and the variety of products and services that it provides.
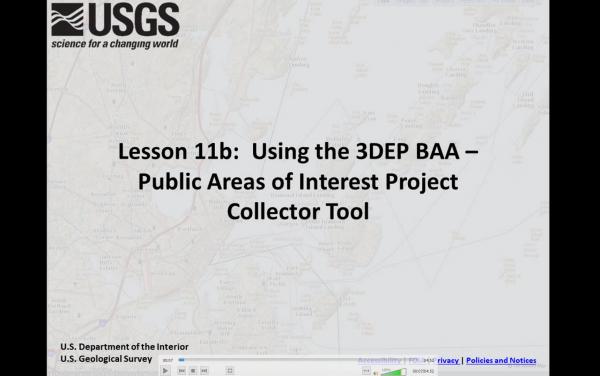
In this lesson you will learn how use the public areas of interest project collection tool to add your lidar project to the Seasketch Demonstration Site for Federal Mapping Data Acquisition Coordination.
In this lesson you will learn how use the public areas of interest project collection tool to add your lidar project to the Seasketch Demonstration Site for Federal Mapping Data Acquisition Coordination.
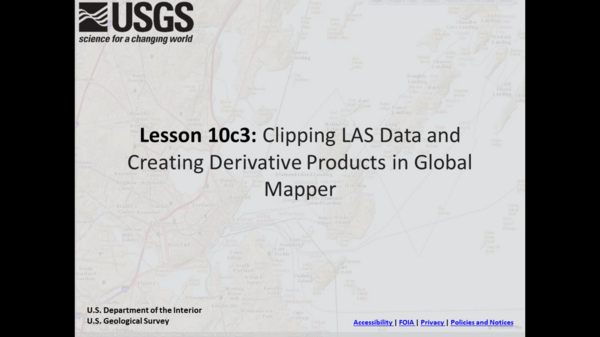
By the end of this lesson, you will be able to load multiple LAS files, define and use a polygon area of interest to subset LAS files, and generate and export raster surfaces and contours derived from the LiDAR point cloud.
By the end of this lesson, you will be able to load multiple LAS files, define and use a polygon area of interest to subset LAS files, and generate and export raster surfaces and contours derived from the LiDAR point cloud.
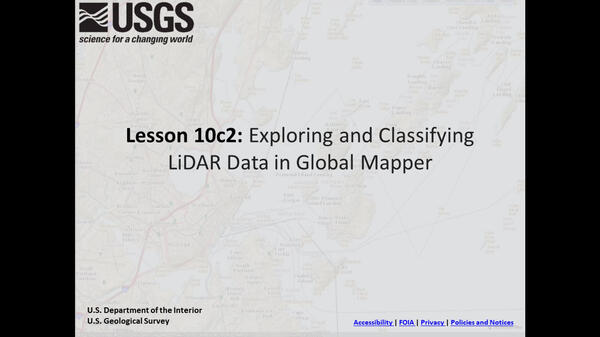
This lesson will cover additional LiDAR point cloud processing and analysis features in Global Mapper.
This lesson will cover additional LiDAR point cloud processing and analysis features in Global Mapper.
This video provides basic information about the HydroLink Tool to get you up and running with the basic tool capabilities.
This video provides basic information about the HydroLink Tool to get you up and running with the basic tool capabilities.
LP360 is a family of tools for visualizing, manipulating and editing point cloud data and are offered both as an extension for ArcGIS, and as a standalone desktop platform for Window.
LP360 is a family of tools for visualizing, manipulating and editing point cloud data and are offered both as an extension for ArcGIS, and as a standalone desktop platform for Window.
Global Mapper is a software package for visualizing, analyzing, manipulating, and exporting a wide variety of GIS data. By the end of this lesson, you will be able to open LiDAR point cloud LAS data files in Global Mapper, turn on all or specific LiDAR point Classes, visualize the data in 2-D and 3-D, and change the visualization using toolbar functions.
Global Mapper is a software package for visualizing, analyzing, manipulating, and exporting a wide variety of GIS data. By the end of this lesson, you will be able to open LiDAR point cloud LAS data files in Global Mapper, turn on all or specific LiDAR point Classes, visualize the data in 2-D and 3-D, and change the visualization using toolbar functions.
This lesson will provide a brief overview of the National Hydrography Dataset, Watershed Boundary Dataset and 3D Elevation program. This lesson will also discuss NHDPlus and introduce NHDPlus high resolution and tell you how to get the data.
This lesson will provide a brief overview of the National Hydrography Dataset, Watershed Boundary Dataset and 3D Elevation program. This lesson will also discuss NHDPlus and introduce NHDPlus high resolution and tell you how to get the data.
This lesson will provide a brief overview of the National Hydrography Dataset (NHD), describe what features make up NHD, show how NHD functions as a national hydrographic network, and provide information on accessing data and additional resources.
This lesson will provide a brief overview of the National Hydrography Dataset (NHD), describe what features make up NHD, show how NHD functions as a national hydrographic network, and provide information on accessing data and additional resources.
The following series of lessons will cover how to use LiDAR data in the ArcGIS Pro environment. In this lesson, we will provide the user with an overview of how to load and view lidar files in the standard LAS format in both 2D and 3D.
The following series of lessons will cover how to use LiDAR data in the ArcGIS Pro environment. In this lesson, we will provide the user with an overview of how to load and view lidar files in the standard LAS format in both 2D and 3D.
In this lesson, we will describe the Watershed Boundary Dataset, show how the data are organized, and explain how the data are improved and maintained. Finally, we will show how the data can be accessed by download and as a map service.
In this lesson, we will describe the Watershed Boundary Dataset, show how the data are organized, and explain how the data are improved and maintained. Finally, we will show how the data can be accessed by download and as a map service.
Related Content
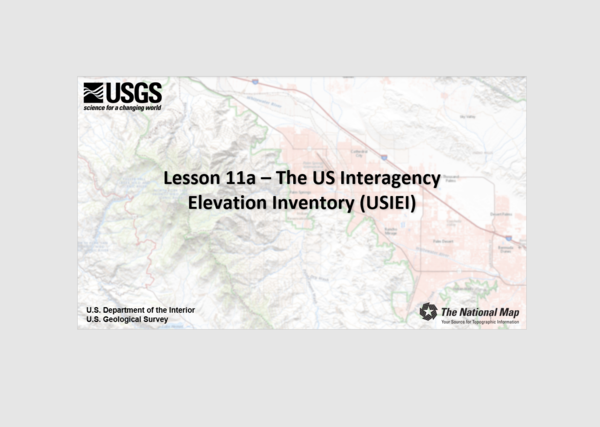
The US Interagency Elevation Inventory, or USIEI, is a nationwide listing of known publicly available high-accuracy topographic and bathymetric source elevation data for the United States and its territories. The inventory provides a single resource for information about all known completed and in-progress broad-area public domain elevation data.
The US Interagency Elevation Inventory, or USIEI, is a nationwide listing of known publicly available high-accuracy topographic and bathymetric source elevation data for the United States and its territories. The inventory provides a single resource for information about all known completed and in-progress broad-area public domain elevation data.
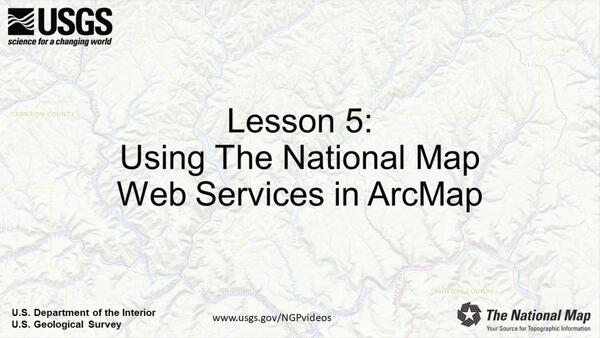
In this lesson, we will provide an overview and demonstrate how to use The National Map’s web services in a mapping application. Although downloading data from The National Map is a popular option for many users, there are numerous situations where it may be preferable to simply call TNM web services into your mapping application.
In this lesson, we will provide an overview and demonstrate how to use The National Map’s web services in a mapping application. Although downloading data from The National Map is a popular option for many users, there are numerous situations where it may be preferable to simply call TNM web services into your mapping application.
This lesson will cover Stream Flow Estimates in NHDPlus High Resolution. Enhanced Runoff Method, or EROM is the method used to compute estimates of the mean annual flow for the NHDPlus flowline features in the NHDPlus HR network.
This lesson will cover Stream Flow Estimates in NHDPlus High Resolution. Enhanced Runoff Method, or EROM is the method used to compute estimates of the mean annual flow for the NHDPlus flowline features in the NHDPlus HR network.
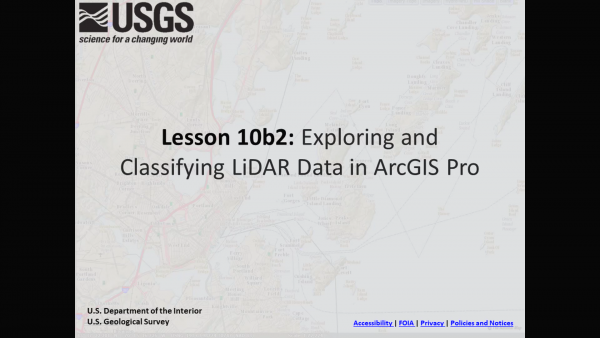
This lesson will cover additional lidar point cloud processing and analysis features in ArcGIS Pro. If necessary, please review lesson 10b1 for guidance with importing LAS files, filtering, and visualizing lidar point cloud data in ArcGIS Pro.
This lesson will cover additional lidar point cloud processing and analysis features in ArcGIS Pro. If necessary, please review lesson 10b1 for guidance with importing LAS files, filtering, and visualizing lidar point cloud data in ArcGIS Pro.
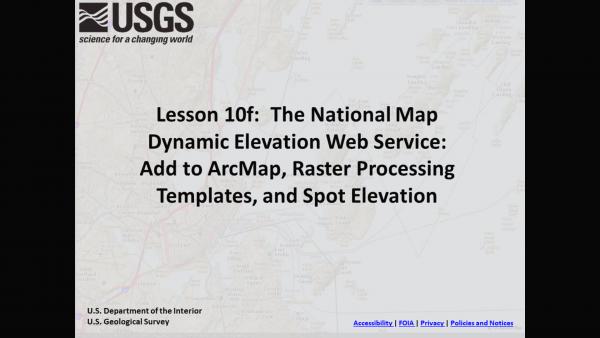
This video tutorial shows how to use the National Map’s 3D Elevation Program (3DEP) Elevation web service in ArcMap. By the end of this lesson, you will be able to add the Dynamic Elevation Web Coverage Service to ArcMap, enable raster processing templates, and retrieve a spot elevation.
This video tutorial shows how to use the National Map’s 3D Elevation Program (3DEP) Elevation web service in ArcMap. By the end of this lesson, you will be able to add the Dynamic Elevation Web Coverage Service to ArcMap, enable raster processing templates, and retrieve a spot elevation.
In this lesson, you will learn about the lidar products available through the 3D Elevation Program (which is abbreviated as “3DEP”). The lesson will introduce 3DEP, discuss the lidar data available, and where to find the data.
In this lesson, you will learn about the lidar products available through the 3D Elevation Program (which is abbreviated as “3DEP”). The lesson will introduce 3DEP, discuss the lidar data available, and where to find the data.
In this lesson we will examine a brief history of the USGS topographic map, Introduce US Topo maps and their content, discuss the production and future goals of US Topo, and learn how to access these maps for viewing or printing.
In this lesson we will examine a brief history of the USGS topographic map, Introduce US Topo maps and their content, discuss the production and future goals of US Topo, and learn how to access these maps for viewing or printing.
By the end of this lesson, you will understand the difference between the LAS and LAZ format, know where to download the laszip.exe tool, and how to decompress a LAZ file to a LAS file using laszip.exe. The USGS offers other lessons on using LAS files in ArcGIS Pro, Global Mapper, and LP360.
By the end of this lesson, you will understand the difference between the LAS and LAZ format, know where to download the laszip.exe tool, and how to decompress a LAZ file to a LAS file using laszip.exe. The USGS offers other lessons on using LAS files in ArcGIS Pro, Global Mapper, and LP360.
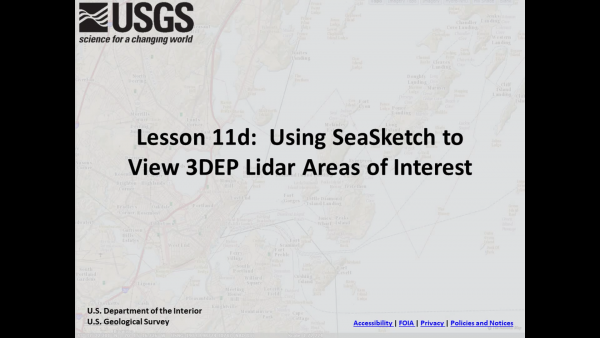
In this lesson you will learn how to zoom to your area of interest and view the footprints of existing and proposed topographic lidar projects.
In this lesson you will learn how to zoom to your area of interest and view the footprints of existing and proposed topographic lidar projects.
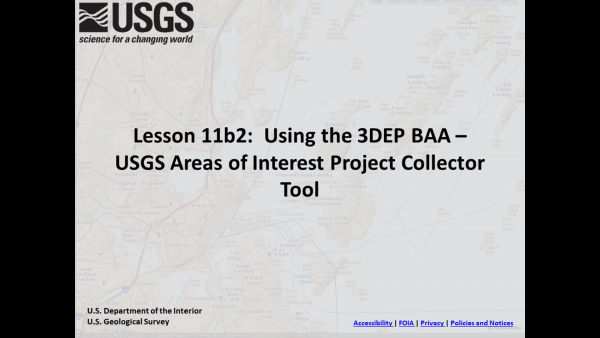
In this lesson you will learn how to use the USGS areas of interest project collection tool to add your lidar project to the Seasketch Demonstration Site for Federal Mapping Data Acquisition Coordination.
In this lesson you will learn how to use the USGS areas of interest project collection tool to add your lidar project to the Seasketch Demonstration Site for Federal Mapping Data Acquisition Coordination.
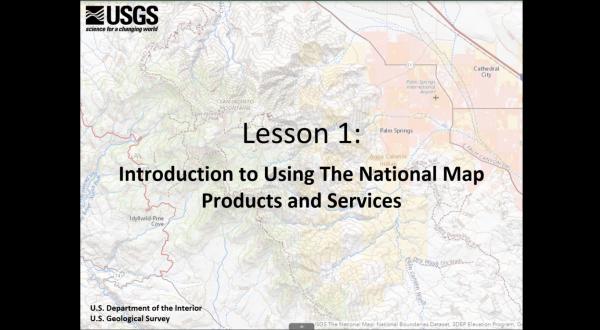
Welcome to the USGS course on Using The National Map Products and Services. This series of videos is focused on The National Map and the variety of products and services that it provides.
Welcome to the USGS course on Using The National Map Products and Services. This series of videos is focused on The National Map and the variety of products and services that it provides.
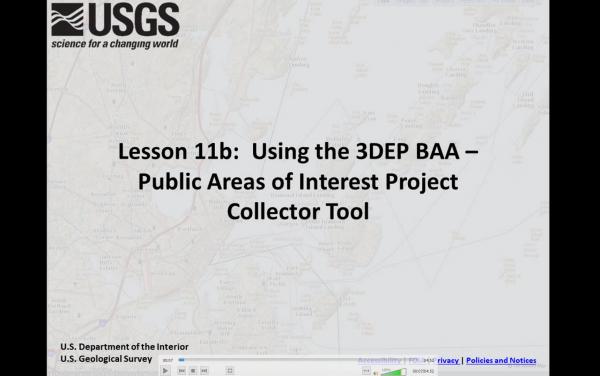
In this lesson you will learn how use the public areas of interest project collection tool to add your lidar project to the Seasketch Demonstration Site for Federal Mapping Data Acquisition Coordination.
In this lesson you will learn how use the public areas of interest project collection tool to add your lidar project to the Seasketch Demonstration Site for Federal Mapping Data Acquisition Coordination.
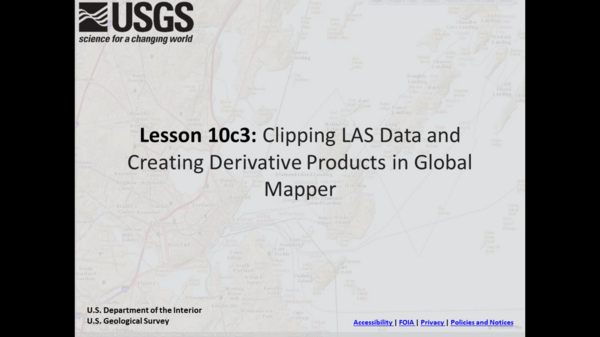
By the end of this lesson, you will be able to load multiple LAS files, define and use a polygon area of interest to subset LAS files, and generate and export raster surfaces and contours derived from the LiDAR point cloud.
By the end of this lesson, you will be able to load multiple LAS files, define and use a polygon area of interest to subset LAS files, and generate and export raster surfaces and contours derived from the LiDAR point cloud.
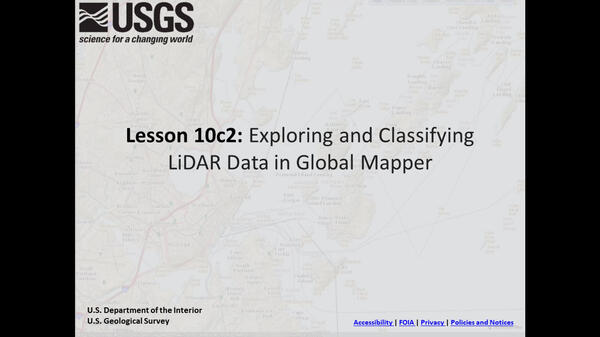
This lesson will cover additional LiDAR point cloud processing and analysis features in Global Mapper.
This lesson will cover additional LiDAR point cloud processing and analysis features in Global Mapper.
This video provides basic information about the HydroLink Tool to get you up and running with the basic tool capabilities.
This video provides basic information about the HydroLink Tool to get you up and running with the basic tool capabilities.
LP360 is a family of tools for visualizing, manipulating and editing point cloud data and are offered both as an extension for ArcGIS, and as a standalone desktop platform for Window.
LP360 is a family of tools for visualizing, manipulating and editing point cloud data and are offered both as an extension for ArcGIS, and as a standalone desktop platform for Window.
Global Mapper is a software package for visualizing, analyzing, manipulating, and exporting a wide variety of GIS data. By the end of this lesson, you will be able to open LiDAR point cloud LAS data files in Global Mapper, turn on all or specific LiDAR point Classes, visualize the data in 2-D and 3-D, and change the visualization using toolbar functions.
Global Mapper is a software package for visualizing, analyzing, manipulating, and exporting a wide variety of GIS data. By the end of this lesson, you will be able to open LiDAR point cloud LAS data files in Global Mapper, turn on all or specific LiDAR point Classes, visualize the data in 2-D and 3-D, and change the visualization using toolbar functions.
This lesson will provide a brief overview of the National Hydrography Dataset, Watershed Boundary Dataset and 3D Elevation program. This lesson will also discuss NHDPlus and introduce NHDPlus high resolution and tell you how to get the data.
This lesson will provide a brief overview of the National Hydrography Dataset, Watershed Boundary Dataset and 3D Elevation program. This lesson will also discuss NHDPlus and introduce NHDPlus high resolution and tell you how to get the data.
This lesson will provide a brief overview of the National Hydrography Dataset (NHD), describe what features make up NHD, show how NHD functions as a national hydrographic network, and provide information on accessing data and additional resources.
This lesson will provide a brief overview of the National Hydrography Dataset (NHD), describe what features make up NHD, show how NHD functions as a national hydrographic network, and provide information on accessing data and additional resources.
The following series of lessons will cover how to use LiDAR data in the ArcGIS Pro environment. In this lesson, we will provide the user with an overview of how to load and view lidar files in the standard LAS format in both 2D and 3D.
The following series of lessons will cover how to use LiDAR data in the ArcGIS Pro environment. In this lesson, we will provide the user with an overview of how to load and view lidar files in the standard LAS format in both 2D and 3D.
In this lesson, we will describe the Watershed Boundary Dataset, show how the data are organized, and explain how the data are improved and maintained. Finally, we will show how the data can be accessed by download and as a map service.
In this lesson, we will describe the Watershed Boundary Dataset, show how the data are organized, and explain how the data are improved and maintained. Finally, we will show how the data can be accessed by download and as a map service.